excerpt: “Improving Primary Care Through Industrial and Systems Engineering”
Helped to create Improving Primary Care Through Industrial and Systems Engineering (I-PrACTISE) collaborative. I-PrACTISE is an educational and research collaborative focused on connecting problems in Primary Care with solutions from Industrial Engineering.
It is a formal partnership between the University of Wisconsin Department of Industrial and Systems Engineering, and the Departments of Family Medicine and Community Health, Medicine and Pediatrics of the UW School of Medicine and Public Health.
I-PrACTISE focuses on applying industrial engineering methods and systems thinking to primary care healthcare settings, aimed at improving patient outcomes while reducing costs and minimizing waste. By doing so, they seek to address some of the challenges facing modern healthcare delivery, which includes rising healthcare costs, limited resources, and burnout.
The goal of I-PrACTISE is to develop a home for cross-disciplinary research to foster development of innovative solutions that involve re-engineering existing clinical workflows and tools.
Vision
The care of patients will be improved and the practice of primary care medicine will become more efficient through new knowledge and techniques created by the collaboration between Industrial Engineering and the primary care specialties.
Mission
Create a home for scholars and clinicians with interest and expertise in industrial engineering and/or primary care to conduct funded projects directed at improving the quality of primary care for patients, clinicians and staff.
Membership
The membership consists of interested UW Faculty from the School of Medicine and Public Health and the Department of Industrial and Systems Engineering as well as interested scholars from other professions and institutions.
I-PrACTISE
excerpt: “Findings from the first I-PrACTISE conference bringing together physicians and engineers.”
—
The first Improving PrimAry Care Through Industrial and Systems Engineering (I-PraCTISE) conference was held at Union South at the University of Wisconsin - Madison in April of 2013.
It was funded by the Agency for Healthcare Research and Quality and co-sponsored by the UW - Madison Departments of Family Medicine and Industrial and Systems Engineering.
A key objective of the first I-PrACTISE conference was to develop a cross-disciplinary research agenda, bringing together engineers and physicians.
I helped to organize themes from across the conference and created this paper to summarize our findings.
Abstract
Primary healthcare is in critical condition with too few students selecting careers, multiple competing demands stressing clinicians, and increasing numbers of elderly patients with multiple health problems. The potential for transdisciplinary research using Industrial and Systems Engineering (ISyE) approaches and methods to study and improve the quality and efficiency of primary care is increasingly recognized. To accelerate the development and application of this research, the National Collaborative to Improve Primary Care through Industrial and Systems Engineering (I-PrACTISE) sponsored an invitational conference in April, 2013 which brought together experts in primary care and ISyE. Seven workgroups were formed, organized around the principles of the Patient Centered Medical Home: Team-Based Care, Coordination and Integration, Health Information Technology (HIT) – Registries and Exchanges, HIT – Clinical Decision Support and Electronic Health Records, Patient Engagement, Access and Scheduling, and Addressing All Health Needs. These groups: (A) Explored critical issues from a primary care perspective and ISyE tools and methods that could address these issues; (B) Generated potential research questions; and (C) Described methods and resources, including other collaborations, needed to conduct this research.
excerpt: “Developed a custom text message gateway for businesses to reach their customers.”
In the days prior to wide smartphone adoption it was hard to find deals on meals and drinks as broke college students on the go.
SMS bottlecap logo
In order to enable restaurants and bars to reach out to college age customers Adam Maus and I created a custom text message gateway integrated with an application and website. These businesses could upload information about their menus and weekly specials and then share them with interested customers by sending out a text message blast.
SMS welcome screen
SMS gateway services existed at the time, but they were very expensive (i.e., you had to pay for each text). To avoid paying per text we got an android smartphone and had it serve as the text message router. We had a webservice that would pass information to an app on the smartphone which would then send text messages using its unlimited data and text plan.
SMS messaging screen
Ultimately, while we were technically successful this project didn’t really go anywhere. We were not addressing a pain point that businesses in Madison were experiencing. Students would have benefited, but they weren’t our “customers”. Cautionary tale on doing good customer discovery and working hard to achieve product-market fit. That’s more important than cool technology.
This case competition involved optimizing the operations of an emergency department (ED) using discrete event simulation and operations research tools. The goal was to analyze the Susquehanna Health ED’s current operations and determine the best care delivery model to meet productivity requirements while satisfying staffing and care constraints.
We used a combination of discrete event simulation (FlexSim healthcare software), design of experiments, and mathematical programming to determine the ideal care delivery model. See below for a copy of our winning presentation.
Executive Summary
Susquehanna Health, a four‐hospital, not‐for‐profit health system, has deployed an Emergency Department (ED) Leadership Team to reduce expenses and optimize operations at their flagship hospital, Williamsport Regional Medical Center (WRMC). The Emergency Department has been experiencing pressure from a recently enacted marketing campaign that ensures patients are seen by a provider in 30 minutes or less at two competitor hospitals in the region. This campaign concerns Susquehanna Health because their current average door to provider time is 42.7 minutes with peak times as long as 140 minutes. As a result, 2.8% of their patients are leaving without being seen.
The Susquehanna Health System needs to be competitive in order to face today’s healthcare trends of declining reimbursement, increasingly high debt, and greater focus on outpatient services. The Emergency Department Leadership Team reached out to UW‐Madison’s Industrial & Systems Engineering students to assist them in creating a simulation that will help them improve patient safety, staff productivity, and overall efficiency.
The UW‐Madison Industrial & Systems Engineering students developed a discrete‐event simulation of WRMC Emergency Department’s traditional triage and bed process using FlexSim HC simulation software. Input data consisted of processing time distributions and probabilities supplied from the Emergency Department Leadership Team. To enhance the accuracy of the model, the team also collaborated with physicians at the University of Wisconsin Hospitals and Clinics (UWHC) to gather information on average processing times. Based on best practices in other institutions, simulation models were created to represent the two additional delivery methods: PITT and PITT/Super Fast Track.
After the modeling process was completed the team ran a series of experiments to determine the optimal delivery method and staffing levels. Super Fast Track appeared to be the best delivery system, however the team recommends that this analysis be redone on a more powerful machine. The machine used for modeling was not powerful enough to run the simulation experiments needed for statistical certainty.
The team views this as the first phase of a longer term project. The team will continue to refine the model and run new experiments once a new machine is procured. Collaborators at the UW – Madison, School of Medicine and Public Health, have asked the team to build a second set of models to be used for the UW Health ED.
Patient throughput time in the emergency department is a critical metric affecting patient satisfaction and service efficiency. We performed a retrospective analysis of electronic medical record (EMR) derived data to evaluate the effectiveness of multiple modeling techniques in predicting throughput times for patient encounters in an academic emergency department (ED). Analysis was conducted using various modeling techniques and on differing amounts of information about each patient encounter. We hypothesized that more comprehensive and inclusive models would provide greater predictive power.
Methods
Retrospective medical record review was performed on consecutive patients at a single, academic, university-based ED. Data were extracted from an EMR derived dataset. All patients who presented from January 1, 2011 to December 31, 2013 and met inclusion criteria were included in the analysis. The data were then partitioned into two sets: one for developing models (training) and a second for analyzing the predictive power of these models (testing). The Table lists model types used. The primary outcome measured was the ability of the trained models to accurately predict the throughput times of test data, measured in terms of mean absolute error (MAE). Secondary outcomes were R2 and mean squared error (MSE). Model factors included a mix of patient specific factors such as triage vital signs, age, chief complaint; factors representing the state of the ED such as census and running average throughput time; and timing factors such as time of day, day of week, and month. The most comprehensive models included a total of 29 distinct factors.
Results
Of the 134,194 patients that were seen in the 3-year period of the study 128,252 met the inclusion criteria; the mean throughput time was 183.327 min (SD 1⁄4 98.447 min). Compared to using a single average throughput time as a naïve model (MAE 1⁄4 80.801 min), univariate models provided improved predictive abilities. More sophisticated models, using machine learning methods and including all available factors provided greater predictive power with the lowest MAE achieved at 73.184 min.
Conclusion
We have demonstrated that including information about incoming patients and the state of the ED at the time of an arrival can aid in the prediction of individual patients’ throughput times. The Multiple Linear Regression model, including all available factors, had the highest predictive accuracy, reducing mean absolute error by over 9% compared to the naïve model. While this represents an improvement in the current state of the art, we believe there is room for further work to generate high quality individual patient predictions. More sophisticated models based on ED workflows may lead to greater predictive power to prospectively estimate patient throughput times at arrival.
Arena
excerpt: “Discrete event simulation and operations modeling for undergrads.”
—
I developed an online course to introduce the Arena simulation application. Arena is a discrete event simulation tool that is widely used throughout the field of industrial engineering. Despite its frequent use and inclusion in undergraduate curicula it is often not well understood by students. This is due to a lack of high quality training materials.
I taught an in-person simulation lab (ISyE 321) and assisted in teaching a theory of simulation course (ISyE 320) with Dr. Laura Albert in 2015 at the University of Wisconsin. During this time I developed a series of modules to show off the functionality of Arena. I subsequently recorded these modules and developed a free online course that is on youtube.
Here’s the first video in the online Arena course that I developed:
I also developed accompanying presentation slides, exercises, and Arena files. If you are interested in accessing these materials please contact me.
File: 2016-02-13-Cherry-Picking.md
Creation Date: “Sat, 27 Jul 2024 12:20:28 +0000”
—
title: “Cherry Picking Patients: Examining the Interval Between Patient Rooming and Resident Self-assignment”
categories:
Blog
Research
tags:
Blog
Research
machine learning
artificial intelligence
healthcare
medicine
electronic health record
excerpt: “Investigating the impact of chief complaints on resident physician self-assignment times in emergency departments.”
—
Study titled “Cherry Picking Patients: Examining the Interval Between Patient Rooming and Resident Self-assignment”. We aimed to evaluate the association between patient chief complaint and the time interval between patient rooming and resident physician self-assignment (“pickup time”). The team hypothesized that significant variation in pickup time would exist based on chief complaint, thereby uncovering resident preferences in patient presentations.[^1]
We aimed to evaluate the association between patient chief complaint and the time interval between patient rooming and resident physician self-assignment (“pickup time”). We hypothesized that significant variation in pickup time would exist based on chief complaint, thereby uncovering resident preferences in patient presentations.
Methods
A retrospective medical record review was performed on consecutive patients at a single, academic, university-based emergency department with over 50,000 visits per year. All patients who presented from August 1, 2012, to July 31, 2013, and were initially seen by a resident were included in the analysis. Patients were excluded if not seen primarily by a resident or if registered with a chief complaint associated with trauma team activation. Data were abstracted from the electronic health record (EHR). The outcome measured was “pickup time,” defined as the time interval between room assignment and resident self-assignment. We examined all complaints with >100 visits, with the remaining complaints included in the model in an “other” category. A proportional hazards model was created to control for the following prespecified demographic and clinical factors: age, race, sex, arrival mode, admission vital signs, Emergency Severity Index code, waiting room time before rooming, and waiting room census at time of rooming.
Results
Of the 30,382 patients eligible for the study, the median time to pickup was 6 minutes (interquartile range = 2–15 minutes). After controlling for the above factors, we found systematic and significant variation in the pickup time by chief complaint, with the longest times for patients with complaints of abdominal problems, numbness/tingling, and vaginal bleeding and shortest times for patients with ankle injury, allergic reaction, and wrist injury.
Conclusions
A consistent variation in resident pickup time exists for common chief complaints. We suspect that this reflects residents preferentially choosing patients with simpler workups and less perceived diagnostic ambiguity. This work introduces pickup time as a metric that may be useful in the future to uncover and address potential physician bias. Further work is necessary to establish whether practice patterns in this study are carried beyond residency and persist among attendings in the community and how these patterns are shaped by the information presented via the EHR.
[^1]: Patterson, B. W., Batt, R. J., Wilbanks, M. D., Otles, E., Westergaard, M. C., & Shah, M. N. (2018). Cherry Picking Patients: Examining the Interval Between Patient Rooming and Resident Self-assignment. Academic Emergency Medicine, 25(7), 742-751.
operations research
excerpt: “Using machine learning to predict the likelihood of inpatient admission at the time of patient triage in emergency departments.”
—
“Forecasting Emergency Department Patient Admissions Utilizing Machine Learning” was a clinical abstract submitted to Academic Emergency Medicine. In this study, we aimed to predict the need for admission at the time of patient triage utilizing data already available in the electronic health record (EHR). We performed a retrospective analysis of EHR-derived data to evaluate the effectiveness of machine learning techniques in predicting the likelihood of admission for patient encounters in an academic emergency department. We hypothesized that more comprehensive & inclusive models would provide greater predictive power.
Multiple studies have identified inpatient bed availability as a key metric for Emergency Department
operational performance. Early planning for patient admissions may allow for optimization of hospital resources.
Objectives
Our study aimed to predict the need for admission at the time of patient triage utilizing data already
available in the electronic health record (EHR). We performed a retrospective analysis of EHR derived data to evaluate the effectiveness of machine learning techniques in predicting the likelihood of admission for patient encounters in an academic emergency department. We hypothesized that more comprehensive & inclusive models would provide greater predictive power.
Methods
All patients who presented from 1/1/2012 to 12/31/2013 and met inclusion criteria were included in the analysis. The data were then partitioned into two sets for training and testing. The primary outcome measured was the ability of the trained models to discern the future admission status of an encounter, measured in terms of area under the receiver operator curve (ROC AUC). A secondary outcome was accuracy (ACC). Model features included a mix of patient specific factors (demographics, triage vital signs, visit and chief complaint history), the state of the ED (census and other performance metrics); and timing factors (time of day, etc.). The most comprehensive models included 682 variables, encoding 328 features, aggregated into 3 feature groups.
Results
Our final analysis included 91,060 patient encounters. 28,838 (31.7%) of these encounters resulted in an
inpatient admission. Compared to using a naïve model, single feature group models provided improved predictive abilities (1.8% - 50.8% improvement in ROC AUC), see figure for details. More sophisticated models, including all available feature groups provided greater predictive power with the greatest achieved at ROC AUC score of 0.756.
Conclusion
We have demonstrated that including information about incoming patients and the state of the ED at the
time of triage can aid in the prediction of individual patients’ likelihood of admission. More sophisticated models using claims, weather, and social media data may lead to greater predictive power to prospectively estimate patient admission likelihood at arrival.
industrial engineering
excerpt: “Exploring the potential benefits and challenges of integrating ML into primary care.”
—
I had the opportunity to give a talk titled “Primary Care & Predictive Analytics” as a part of the I-PrACTISE colloquia series. We discussed artificial intelligence/machine learning and their applications in medicine, with a particular focus on primary care. In the presentation, I aimed to demystify machine learning, discuss its potential benefits in healthcare, and address the challenges associated with implementing these cutting-edge techniques.
What is Machine Learning?
Machine learning is a discipline that explores the construction and study of algorithms that can learn from data. These algorithms improve their performance at specific tasks as they gain experience, which is often measured in terms of data. In my talk, I explained the concept of machine learning by drawing parallels between training an algorithm and training an undergraduate. Just as we teach undergraduates general concepts and facts that they then synthesize and apply to specific situations, we train algorithms using data to improve their performance at a given task.
Applications in Medicine and Primary Care
Machine learning has the potential to revolutionize the field of medicine, and primary care is no exception. By leveraging vast amounts of data, we can train algorithms to predict patient outcomes, diagnose conditions more accurately, and identify potential treatment options. For example, we could use machine learning to analyze tumor samples and train a model to evaluate new samples, helping doctors make more informed decisions about cancer diagnosis and treatment.
Challenges and Considerations
Despite its potential, there are several challenges to integrating machine learning into healthcare, particularly in sensitive areas like primary care. One of the key issues I addressed in my talk is the need for collaboration between engineers, computer scientists, statisticians, and healthcare professionals to ensure that these advanced techniques are applied responsibly and effectively.
Additionally, it is crucial to consider the human factors involved in implementing machine learning in healthcare settings. Understanding how healthcare providers interact with and use these algorithms is essential to ensuring their successful integration into medical practice.
Looking Ahead
As we continue to explore the potential of machine learning in primary care and the broader medical field, it is vital to remain focused on responsible development and implementation. By collaborating across disciplines and considering the human factors involved, we can work towards harnessing the power of machine learning to improve patient outcomes and revolutionize healthcare.
Video
File: 2016-07-01-Metastar-Community-Pharmacy_Initiative.md
Creation Date: “Sat, 27 Jul 2024 12:20:28 +0000”
—
title: “A community pharmacy initiative to decrease hospital readmissions by increasing patient adherence and competency of therapy”
categories:
Blog
Research
tags:
Blog
Research
medicine
healthcare
industrial engineering
health system engineering
data science
quality improvement
excerpt: “Pharmacy based interventions may help to reduce hospital admissions.”
—
While working as the lead data scientist at MetaStar I helped to analyze the impact of a community pharmacy based intervention to reduce the rate of hospital admissions and readmissions. Patients enrolled in the intervention had the community pharamcy deliver medications to the homes of patients and educate them as well. We found that enrolling patients in the program reduced their rate of admissions.
Abstract
Background
Direct pharmacist care has been associated with substantial reduction in hospital admission and readmission rates and other positive outcomes, as compared with the absence of such care.
Objective
To decrease readmissions for community pharmacy patients through a program of improved medication packaging, delivery and patient education.
Design
Comparison of the number of admissions and readmissions for each patient enrolled in the program, comparing the time elapsed since enrollment with the equivalent period prior to enrollment.
Setting
A community pharmacy in Kenosha, Wisconsin.
Patients
Medicare beneficiaries served by the community pharmacy conducting the intervention. This includes 263 patients, 167 of which are Medicare beneficiaries, who have been placed in the intervention group as of June 2016.
Intervention
A voluntary program to package medications according to patient-specific characteristics and physician orders, to deliver medication to patients’ homes, and to educate and follow up with patients regarding problems with adherence.
Measurements
Hospital admissions and readmissions post-enrollment as compared with the equivalent pre-enrollment period.
Results
An analysis that limits the study period to a year centered on the patient’s enrollment date in the PACT intervention found a highly statistically significant (p < 0.01) reduction in admissions. An analysis that included the entire duration of the patient’s enrollment in PACT also found a statistically significant (p < 0.001) reduction in admissions. However, neither analytic technique found a statistically significant reduction in readmissions (p=0.2 and 0.1 respectively).
Limitations
That the study was unable to show a decrease in readmissions to accompany the decrease in admissions may be due to the success of the intervention in decreasing the denominator as well as the numerator of the readmissions measure. In addition, the study has not stratified for changes in the intervention over time, and for differences in patient characteristics or outcomes other than admissions and readmissions.
File: 2016-08-01-INFORMS-in-the-News.md
Creation Date: “Sat, 27 Jul 2024 12:20:28 +0000”
—
title: “Quoted in INFORMS in the News”
categories:
Blog
Press
tags:
Blog
Press
excerpt: “Starting a new INFORMS student chapter.”
—
Over the course of the 2015-2016 school year I worked with several other students to start a student chapter of INFORMS at UW - Madison. After putting together bylaws and dealing with red tape we got the new student organization started. Additionally, was quoted in INFORMS in the News regarding setting up the University of Wisconsin student INFORMS chapter.
File: 2016-09-19-Impact-of-ED-Census-on-Admission.md
Creation Date: “Sat, 27 Jul 2024 12:20:28 +0000”
—
title: “The Impact of ED Census on the Decision to Admit”
categories:
Blog
Research
tags:
Blog
Research
machine learning
artificial intelligence
healthcare
medicine
electronic health record
operations research
health system engineering
excerpt: “Emergency Department disposition decisions are influenced not only by objective measures of a patient’s disease state, but also by census.”
—
Jillian K. Gorski, Robert J. Batt, PhD, myself, Manish N. Shah, MD MPH, Azita G. Hamedani MD, MPH, MBA, and Brian W. Patterson MD, MPH, studied the impact of emergency department (ED) census on disposition decisions made by ED physicians. Our findings reveal that disposition decisions in the ED are not solely influenced by objective measures of a patient’s condition, but are also affected by workflow-related concerns.
The retrospective analysis involved 18 months of all adult patient encounters in the main ED at an academic tertiary care center. The results demonstrated that both waiting room census and physician load census were significantly associated with an increased likelihood of patient admission. This highlights the need to consider workflow-related factors when making disposition decisions, in order to ensure optimal patient care and resource allocation in emergency departments.
Abstract
Objective
We evaluated the effect of emergency department (ED) census on disposition decisions made by ED physicians.
Methods
We performed a retrospective analysis using 18 months of all adult patient encounters seen in the main ED at an academic tertiary care center. Patient census information was calculated at the time of physician assignment for each individual patient and included the number of patients in the waiting room (waiting room census) and number of patients being managed by the patient’s attending (physician load census). A multiple logistic regression model was created to assess the association between these census variables and the disposition decision, controlling for potential confounders including Emergency Severity Index acuity, patient demographics, arrival hour, arrival mode, and chief complaint.
Results
A total of 49,487 patient visits were included in this analysis, of whom 37% were admitted to the hospital. Both census measures were significantly associated with increased chance of admission; the odds ratio (OR) per patient increase for waiting room census was 1.011 (95% confidence interval [CI] = 1.001 to 1.020), and the OR for physician load census was 1.010 (95% CI = 1.002 to 1.019). To put this in practical terms, this translated to a modeled rise from 35.3% to 40.1% when shifting from an empty waiting room and zero patient load to a 12-patient wait and 16-patient load for a given physician.
Conclusion
Waiting room census and physician load census at time of physician assignment were positively associated with the likelihood that a patient would be admitted, controlling for potential confounders. Our data suggest that disposition decisions in the ED are influenced not only by objective measures of a patient’s disease state, but also by workflow-related concerns.
File: 2016-10-01-Cues-for-PE-diagnosis-in-the-ED.md
Creation Date: “Sat, 27 Jul 2024 12:20:28 +0000”
—
title: “Cues for PE Diagnosis in the Emergency Department: A Sociotechnical Systems Approach for Clinical Decision Support”
categories:
Blog
Research
tags:
Blog
Research
emergency medicine
medicine
healthcare
health system engineering
human factors engineering
excerpt: “American Medical Informatics Association Annual Symposium abstract focused on pulmonary embolism diagnosis.”
—
Pulmonary embolism (PE) diagnosis presents a significant challenge for emergency department (ED) physicians, as both missed or delayed diagnosis and overtesting can have serious consequences for patients. The implementation of health information technology, such as clinical decision support systems, has the potential to mitigate diagnostic errors and enhance the overall diagnostic process. However, to achieve this, the technology must be practical, user-friendly, and seamlessly integrate into clinical workflows. This calls for a sociotechnical systems approach to understand the cues involved in the PE diagnosis process and how they relate to the information available in electronic health records (EHRs).
In this study, we sought to comprehend the cues in the PE diagnosis process within the ED sociotechnical system and compare them to the information found in the EHR. The objective was to establish design requirements for clinical decision support for PE diagnosis in the ED.
Abstract
Pulmonary embolus (PE) is among the most challenging diagnoses made in the emergency department (ED). While missed or delayed diagnosis of PE is a major problem in the ED1, overtesting, which subjects patients to harm from radiation, overdiagnosis, and increased cost, is also a concern. Health information technology, such as clinical decision support, has the potential to reduce diagnostic errors and support the diagnostic process. However, this requires that the technology be useful and usable, and fit within the clinical workflow, providing justification for a sociotechnical systems approach. The purpose of this study is to understand cues in the PE diagnosis process in the ED sociotechnical system and to compare these cues to the information available in the EHR. This will help in defining design requirements for a clinical decision support for PE diagnosis in the ED.
Using the Critical Decision Method, we interviewed 16 attending physicians and residents in three EDs of two academic medical centers and one community hospital. The total duration of the interviews was over 12 hours. Using an iterative qualitative content analysis, we identified 4 categories of cues: (1) patient signs and symptoms (e.g., leg swelling, chest pain), (2) patient risk factors (e.g., immobilization, surgery or trauma, cancer), (3) explicit risk scoring (e.g., PERC), and (4) clinical judgment. We then mapped these cues to information available in the EHR at one of the participating hospitals. About 80-90% of the cues may be available in the EHR; many of them rely on the physical exam and information obtained by talking to the patient. This finding underlines the need to identify the various roles involved in obtaining, documenting and reviewing the information that informs the PE diagnostic process. The PE diagnostic process in the ED is distributed across multiple roles, individuals and technologies in a sometimes chaotic and often busy physical and organizational environment.
Its part of the World Health Organization’s technical series on safer primary care, and has a particular focus on human factors. This report highlights the crucial role that human factors play in ensuring patient safety, improving the quality of care, and optimizing the overall efficiency of primary care systems. By understanding the interaction between humans, systems, and technologies, healthcare organizations can implement more effective strategies to reduce errors, enhance communication, and ultimately improve patient outcomes.
This monograph describes what “human factors” are and what relevance this approach has for improving safety in primary care. This section defines human factors. The next sections outline some of the key human factors’ issues in primary care and the final sections explore potential practical solutions for safer primary care.
File: 2020-05-12-Faster-than-COVID.md
Creation Date: “Sat, 27 Jul 2024 12:20:28 +0000”
—
title: “Faster than COVID: a computer model that predicts the disease’s next move”
categories:
Blog
Press
tags:
Blog
Press
covid
artificial intelligence
machine learning
early warning system
Michigan Engineering News covered our work on the M-CURES COVID deterioration model that I helped to develop and led the implementation of. Read the article here.
File: 2020-05-29-AADL-Friday-Night-AI.md
Creation Date: “Sat, 27 Jul 2024 12:20:28 +0000”
—
title: “Ann Arbor District Library - Friday Night AI: AI and COVID-19”
categories:
Blog
Talk
tags:
medicine
healthcare
covid
machine learning
artificial intelligence
operations research
Virtual panel discussion on how artificial intelligence could guide the response to the coronavirus outbreak. Hosted by the Ann Arbor District Library. Panel included speakers from across the Michigan AI and Michigan Medicine.
File: 2020-05-30-its-time-to-bring-human-factors-to-primary-care.md
Creation Date: “Sat, 27 Jul 2024 12:20:28 +0000”
—
title: “It’s time to bring human factors to primary care policy and practice”
categories:
Primary health care is a complex, highly personal, and non-linear process. Care is often sub-optimal and professional burnout is high. Interventions intended to improve the situation have largely failed. This is due to a lack of a deep understanding of primary health care. Human Factors approaches and methods will aid in understanding the cognitive, social and technical needs of these specialties, and in designing and testing proposed innovations. In 2012, Ben-Tzion Karsh, Ph.D., conceived a transdisciplinary conference to frame the opportunities for research human factors and industrial engineering in primary care. In 2013, this conference brought together experts in primary care and human factors to outline areas where human factors methods can be applied. The results of this expert consensus panel highlighted four major research areas: Cognitive and social needs, patient engagement, care of community, and integration of care. Work in these areas can inform the design, implementation, and evaluation of innovations in Primary Care. We provide descriptions of these research areas, highlight examples and give suggestions for future research.
————————
File: 2020-09-23-UM-Precision-Health-Symposium.md
Creation Date: “Sat, 27 Jul 2024 12:20:28 +0000”
—
title: “UMich Precision Health Symposium: Prediction & Prevention - Powering Precision Health”
categories:
Blog
Talk
tags:
medicine
healthcare
precision health
machine learning
artificial intelligence
operations research
Virtual panel discussion on precison health. A video segment from the 2020 University of Michigan Precision Health Virtual Symposium.
Precision Health Data Analytics & IT workgroup held an onboarding session for Engineering students who could use Precision Health tools and resources for their classes and research. I provided a technical demonstration on how to find and query the database through the sql server.
Was featured in the University of Michigan Medical Scientist Training Program recruiting video.
The MSTP at Michigan prepares physician scientists for careers in academic medicine with a focus on biomedical research. More than just an M.D. and Ph.D. spliced together, our program offers comprehensive support and guidance, integrating academic excellence and flexibility to help you reach your career goals.
File: 2021-07-21-External-Validation-of-a-Widely-Implemented-Proprietary-Sepsis-Prediction-Model-in-Hospitalized-Patients.md
Creation Date: “Sat, 27 Jul 2024 12:20:28 +0000”
—
title: “External Validation of a Widely Implemented Proprietary Sepsis Prediction Model in Hospitalized Patients”
categories:
How accurately does the Epic Sepsis Model, a proprietary sepsis prediction model implemented at hundreds of US hospitals, predict the onset of sepsis?
Findings
In this cohort study of 27 697 patients undergoing 38 455 hospitalizations, sepsis occurred in 7% of the hosptalizations. The Epic Sepsis Model predicted the onset of sepsis with an area under the curve of 0.63, which is substantially worse than the performance reported by its developer.
Meaning
This study suggests that the Epic Sepsis Model poorly predicts sepsis; its widespread adoption despite poor performance raises fundamental concerns about sepsis management on a national level.
Abstract
Importance
The Epic Sepsis Model (ESM), a proprietary sepsis prediction model, is implemented at hundreds of US hospitals. The ESM’s ability to identify patients with sepsis has not been adequately evaluated despite widespread use.
Objective
To externally validate the ESM in the prediction of sepsis and evaluate its potential clinical value compared with usual care.
Design, Setting, and Participants
This retrospective cohort study was conducted among 27 697 patients aged 18 years or older admitted to Michigan Medicine, the academic health system of the University of Michigan, Ann Arbor, with 38 455 hospitalizations between December 6, 2018, and October 20, 2019.
Exposure
The ESM score, calculated every 15 minutes.
Main Outcomes and Measures
Sepsis, as defined by a composite of (1) the Centers for Disease Control and Prevention surveillance criteria and (2) International Statistical Classification of Diseases and Related Health Problems, Tenth Revision diagnostic codes accompanied by 2 systemic inflammatory response syndrome criteria and 1 organ dysfunction criterion within 6 hours of one another. Model discrimination was assessed using the area under the receiver operating characteristic curve at the hospitalization level and with prediction horizons of 4, 8, 12, and 24 hours. Model calibration was evaluated with calibration plots. The potential clinical benefit associated with the ESM was assessed by evaluating the added benefit of the ESM score compared with contemporary clinical practice (based on timely administration of antibiotics). Alert fatigue was evaluated by comparing the clinical value of different alerting strategies.
Results
We identified 27 697 patients who had 38 455 hospitalizations (21 904 women [57%]; median age, 56 years [interquartile range, 35-69 years]) meeting inclusion criteria, of whom sepsis occurred in 2552 (7%). The ESM had a hospitalization-level area under the receiver operating characteristic curve of 0.63 (95% CI, 0.62-0.64). The ESM identified 183 of 2552 patients with sepsis (7%) who did not receive timely administration of antibiotics, highlighting the low sensitivity of the ESM in comparison with contemporary clinical practice. The ESM also did not identify 1709 patients with sepsis (67%) despite generating alerts for an ESM score of 6 or higher for 6971 of all 38 455 hospitalized patients (18%), thus creating a large burden of alert fatigue.
Conclusions and Relevance
This external validation cohort study suggests that the ESM has poor discrimination and calibration in predicting the onset of sepsis. The widespread adoption of the ESM despite its poor performance raises fundamental concerns about sepsis management on a national level.
————————
File: 2021-07-21-STAT-News-Epic-sepsis.md
Creation Date: “Sat, 27 Jul 2024 12:20:28 +0000”
—
title: “STAT News: A popular algorithm to predict sepsis misses most cases and sends frequent false alarms, study finds”
categories:
File: 2021-07-22-The-Verge-A-hospital-algorithm-designed-to-predict-a-deadly-condition-misses-most-cases.md
Creation Date: “Sat, 27 Jul 2024 12:20:28 +0000”
—
title: “The Verge: A hospital algorithm designed to predict a deadly condition misses most cases”
categories:
File: 2021-07-26-The-Washington-Post-A-hospital-algorithm-designed-to-predict-a-deadly-condition-misses-most-cases copy.md
Creation Date:
—
title: “The Washington Post: Sepsis prediction tool used by hospitals misses many cases, study says. Firm that developed the tool disputes those findings.”
categories:
Our 2021 Machine Learning for Healthcare Conference paper! It discusses a special kind of dataset shift that is particularly pervasive and pernicious when developing and implementing ML/AI models for use in healthcare. Here’s a link to the Mind the Performance Gap paper that I authored with Jeeheh Oh, Benjamin Li, Michelle Bochinski, Hyeon Joo, Justin Ortwine, Erica Shenoy, Laraine Washer, Vincent B. Young, Krishna Rao, and Jenna Wiens.
Abstract
Once integrated into clinical care, patient risk stratification models may perform worse com- pared to their retrospective performance. To date, it is widely accepted that performance will degrade over time due to changes in care processes and patient populations. However, the extent to which this occurs is poorly understood, in part because few researchers re- port prospective validation performance. In this study, we compare the 2020-2021 (’20-’21) prospective performance of a patient risk stratification model for predicting healthcare- associated infections to a 2019-2020 (’19-’20) retrospective validation of the same model. We define the difference in retrospective and prospective performance as the performance gap. We estimate how i) “temporal shift”, i.e., changes in clinical workflows and patient populations, and ii) “infrastructure shift”, i.e., changes in access, extraction and transfor- mation of data, both contribute to the performance gap. Applied prospectively to 26,864 hospital encounters during a twelve-month period from July 2020 to June 2021, the model achieved an area under the receiver operating characteristic curve (AUROC) of 0.767 (95% confidence interval (CI): 0.737, 0.801) and a Brier score of 0.189 (95% CI: 0.186, 0.191). Prospective performance decreased slightly compared to ’19-’20 retrospective performance, in which the model achieved an AUROC of 0.778 (95% CI: 0.744, 0.815) and a Brier score of 0.163 (95% CI: 0.161, 0.165). The resulting performance gap was primarily due to in- frastructure shift and not temporal shift. So long as we continue to develop and validate models using data stored in large research data warehouses, we must consider differences in how and when data are accessed, measure how these differences may negatively affect prospective performance, and work to mitigate those differences.
————————
File: 2021-08-01-evaluating-a-widely-implemented-proprietary-deterioration-index-among-inpatients-with-COVID.md
Creation Date: “Sat, 27 Jul 2024 12:20:28 +0000”
—
title: “Evaluating a Widely Implemented Proprietary Deterioration Index Model among Hospitalized Patients with COVID-19”
categories:
Blog
Research
tags:
Blog
Research
COVID
deterioration index
Epic
early warning system
medicine
healthcare
artificial intelligence
machine learning
Annals of the American Thoracic Society. Can be found here.
The Epic Deterioration Index (EDI) is a proprietary prediction model implemented in over 100 U.S. hospitals that was widely used to support medical decision-making during the coronavirus disease (COVID-19) pandemic. The EDI has not been independently evaluated, and other proprietary models have been shown to be biased against vulnerable populations.
Objectives
To independently evaluate the EDI in hospitalized patients with COVID-19 overall and in disproportionately affected subgroups.
Methods
We studied adult patients admitted with COVID-19 to units other than the intensive care unit at a large academic medical center from March 9 through May 20, 2020. We used the EDI, calculated at 15-minute intervals, to predict a composite outcome of intensive care unit–level care, mechanical ventilation, or in-hospital death. In a subset of patients hospitalized for at least 48 hours, we also evaluated the ability of the EDI to identify patients at low risk of experiencing this composite outcome during their remaining hospitalization.
Results
Among 392 COVID-19 hospitalizations meeting inclusion criteria, 103 (26%) met the composite outcome. The median age of the cohort was 64 (interquartile range, 53–75) with 168 (43%) Black patients and 169 (43%) women. The area under the receiver-operating characteristic curve of the EDI was 0.79 (95% confidence interval, 0.74–0.84). EDI predictions did not differ by race or sex. When exploring clinically relevant thresholds of the EDI, we found patients who met or exceeded an EDI of 68.8 made up 14% of the study cohort and had a 74% probability of experiencing the composite outcome during their hospitalization with a sensitivity of 39% and a median lead time of 24 hours from when this threshold was first exceeded. Among the 286 patients hospitalized for at least 48 hours who had not experienced the composite outcome, 14 (13%) never exceeded an EDI of 37.9, with a negative predictive value of 90% and a sensitivity above this threshold of 91%.
Conclusions
We found the EDI identifies small subsets of high-risk and low-risk patients with COVID-19 with good discrimination, although its clinical use as an early warning system is limited by low sensitivity. These findings highlight the importance of independent evaluation of proprietary models before widespread operational use among patients with COVID-19.
————————
File: 2021-08-05-MLHC-Presentation.md
Creation Date: “Sat, 27 Jul 2024 12:20:28 +0000”
—
title: “Machine Learning for Healthcare Conference: Characterizing the Performance Gap”
categories:
Blog
Talk
tags:
medicine
healthcare
machine learning
artificial intelligence
operations research
Jeeheh Oh and I presented our work on dataset shift at the 2021 Machine Learning for Healthcare Conference. This talk briefly summarizes our our conference paper.
Abstract
Once integrated into clinical care, patient risk stratification models may perform worse com- pared to their retrospective performance. To date, it is widely accepted that performance will degrade over time due to changes in care processes and patient populations. However, the extent to which this occurs is poorly understood, in part because few researchers re- port prospective validation performance. In this study, we compare the 2020-2021 (’20-’21) prospective performance of a patient risk stratification model for predicting healthcare- associated infections to a 2019-2020 (’19-’20) retrospective validation of the same model. We define the difference in retrospective and prospective performance as the performance gap. We estimate how i) “temporal shift”, i.e., changes in clinical workflows and patient populations, and ii) “infrastructure shift”, i.e., changes in access, extraction and transfor- mation of data, both contribute to the performance gap. Applied prospectively to 26,864 hospital encounters during a twelve-month period from July 2020 to June 2021, the model achieved an area under the receiver operating characteristic curve (AUROC) of 0.767 (95% confidence interval (CI): 0.737, 0.801) and a Brier score of 0.189 (95% CI: 0.186, 0.191). Prospective performance decreased slightly compared to ’19-’20 retrospective performance, in which the model achieved an AUROC of 0.778 (95% CI: 0.744, 0.815) and a Brier score of 0.163 (95% CI: 0.161, 0.165). The resulting performance gap was primarily due to in- frastructure shift and not temporal shift. So long as we continue to develop and validate models using data stored in large research data warehouses, we must consider differences in how and when data are accessed, measure how these differences may negatively affect prospective performance, and work to mitigate those differences.
————————
File: 2021-10-11-CHEPS-Seminar.md
Creation Date: “Sat, 27 Jul 2024 12:20:28 +0000”
—
title: “CHEPS Seminar: Engineering Machine Learning for Medicine”
categories:
Blog
Talk
tags:
medicine
healthcare
machine learning
artificial intelligence
operations research
industrial engineering
health system engineering
Invited to give a talk for the 2021 University of Michigan Center for Healthcare Engineering and Patient Safety (CHEPS) fall seminar series. Discussed engineering machine learning for medicine. Gave an overview of the whole healthcare AI/ML lifecycle and discussed it is chockablock with cool industrial & health systems engineering problems.
File: 2021-10-31-Using-NLP-to-Automatically-Assess-Feedback-Quality.md
Creation Date: “Sat, 27 Jul 2024 12:20:28 +0000”
—
title: “Using Natural Language Processing to Automatically Assess Feedback Quality: Findings From 3 Surgical Residencies”
categories:
Learning is markedly improved with high-quality feedback, yet assuring the quality of feedback is difficult to achieve at scale. Natural language processing (NLP) algorithms may be useful in this context as they can automatically classify large volumes of narrative data. However, it is unknown if NLP models can accurately evaluate surgical trainee feedback. This study evaluated which NLP techniques best classify the quality of surgical trainee formative feedback recorded as part of a workplace assessment.
Method
During the 2016–2017 academic year, the SIMPL (Society for Improving Medical Professional Learning) app was used to record operative performance narrative feedback for residents at 3 university-based general surgery residency training programs. Feedback comments were collected for a sample of residents representing all 5 postgraduate year levels and coded for quality. In May 2019, the coded comments were then used to train NLP models to automatically classify the quality of feedback across 4 categories (effective, mediocre, ineffective, or other). Models included support vector machines (SVM), logistic regression, gradient boosted trees, naive Bayes, and random forests. The primary outcome was mean classification accuracy.
Results
The authors manually coded the quality of 600 recorded feedback comments. Those data were used to train NLP models to automatically classify the quality of feedback across 4 categories. The NLP model using an SVM algorithm yielded a maximum mean accuracy of 0.64 (standard deviation, 0.01). When the classification task was modified to distinguish only high-quality vs low-quality feedback, maximum mean accuracy was 0.83, again with SVM.
Conclusions
To the authors’ knowledge, this is the first study to examine the use of NLP for classifying feedback quality. SVM NLP models demonstrated the ability to automatically classify the quality of surgical trainee evaluations. Larger training datasets would likely further increase accuracy.
————————
File: 2021-11-01-INFORMS-Dynamic-Machine-Learning.md
Creation Date: “Sat, 27 Jul 2024 12:20:28 +0000”
—
title: “INFORMS: Dynamic Machine Learning for Medical Practice”
categories:
Blog
Talk
tags:
medicine
healthcare
machine learning
artificial intelligence
operations research
industrial engineering
health system engineering
INFORMS conference talk focused on dynamic machine learning for medicine. Based on Joint work with Jon Seymour, MD (Peers Health) and Brian Denton PhD (University of Michigan).
Time is a crucial factor of clinical practice. Our work explores the intersection of time and machine learning (ML) in the context of medicine. This presentation will examine the creation, validation, and deployment of dynamic ML models. We discuss dynamic prediction of future work status for patients who have experienced occupational injuries. Methodologically we cover a framework for dynamic prediction health-state prediction that combines a novel data transformation with an appropriate automatically generated deep learning architecture. These projects expand our understanding of how to effectively train and utilize dynamic machine learning models in the service of advancing health.
File: 2021-11-02-Trust-The-AI-You-Decide.md
Creation Date: “Sat, 27 Jul 2024 12:20:28 +0000”
—
title: “Forbes: Trust The AI? You Decide”
categories:
File: 2021-11-19-Quantification-of-Sepsis-Model-Alerts-in-24-US-Hospitals-Before-and-During-the-COVID-19-Pandemic.md
Creation Date: “Sat, 27 Jul 2024 12:20:28 +0000”
—
title: “Quantification of Sepsis Model Alerts in 24 US Hospitals Before and During the COVID-19 Pandemic”
categories:
To validate the performance of a natural language processing (NLP) model in characterizing the quality of feedback provided to surgical trainees.
Design
Narrative surgical resident feedback transcripts were collected from a large academic institution and classified for quality by trained coders. 75% of classified transcripts were used to train a logistic regression NLP model and 25% were used for testing the model. The NLP model was trained by uploading classified transcripts and tested using unclassified transcripts. The model then classified those transcripts into dichotomized high- and low- quality ratings. Model performance was primarily assessed in terms of accuracy and secondary performance measures including sensitivity, specificity, and area under the receiver operating characteristic curve (AUROC).
Setting
A surgical residency program based in a large academic medical center.
Participants
All surgical residents who received feedback via the Society for Improving Medical Professional Learning smartphone application (SIMPL, Boston, MA) in August 2019.
Results
The model classified the quality (high vs. low) of 2,416 narrative feedback transcripts with an accuracy of 0.83 (95% confidence interval: 0.80, 0.86), sensitivity of 0.37 (0.33, 0.45), specificity of 0.97 (0.96, 0.98), and an area under the receiver operating characteristic curve of 0.86 (0.83, 0.87).
Conclusions
The NLP model classified the quality of operative performance feedback with high accuracy and specificity. NLP offers residency programs the opportunity to efficiently measure feedback quality. This information can be used for feedback improvement efforts and ultimately, the education of surgical trainees.
————————
File: 2021-12-02-NLP-to-Estimate-Clinical-Competency-Committee-Ratings.md
Creation Date: “Sat, 27 Jul 2024 12:20:28 +0000”
—
title: “Natural Language Processing to Estimate Clinical Competency Committee Ratings”
categories:
Residency program faculty participate in clinical competency committee (CCC) meetings, which are designed to evaluate residents’ performance and aid in the development of individualized learning plans. In preparation for the CCC meetings, faculty members synthesize performance information from a variety of sources. Natural language processing (NLP), a form of artificial intelligence, might facilitate these complex holistic reviews. However, there is little research involving the application of this technology to resident performance assessments. With this study, we examine whether NLP can be used to estimate CCC ratings.
Design
We analyzed end-of-rotation assessments and CCC assessments for all surgical residents who trained at one institution between 2014 and 2018. We created models of end-of-rotation assessment ratings and text to predict dichotomized CCC assessment ratings for 16 Accreditation Council for Graduate Medical Education (ACGME) Milestones. We compared the performance of models with and without predictors derived from NLP of end-of-rotation assessment text.
Results
We analyzed 594 end-of-rotation assessments and 97 CCC assessments for 24 general surgery residents. The mean (standard deviation) for area under the receiver operating characteristic curve (AUC) was 0.84 (0.05) for models with only non-NLP predictors, 0.83 (0.06) for models with only NLP predictors, and 0.87 (0.05) for models with both NLP and non-NLP predictors.
Conclusions
NLP can identify language correlated with specific ACGME Milestone ratings. In preparation for CCC meetings, faculty could use information automatically extracted from text to focus attention on residents who might benefit from additional support and guide the development of educational interventions.
————————
File: 2021-12-04-Comparative-Assessment-of-a-Machine-Learning-Model-and-Rectal-Swab-Surveillance-to-Predict-Hospital-Onset-Clostridioides-difficile.md
Creation Date: “Sat, 27 Jul 2024 12:20:28 +0000”
—
title: “Comparative Assessment of a Machine Learning Model and Rectal Swab Surveillance to Predict Hospital Onset Clostridioides difficile”
categories:
Shared an overview of my research during the 2021 University of Michigan Department of Industrial and Operations Engineering recruiting weekend.
File: 2021-12-09-Precision-Health-Webinar.md
Creation Date: “Sat, 27 Jul 2024 12:20:28 +0000”
—
title: “Precision Health Webinar: What Clinicians Need to Know when Using AI”
categories:
Blog
Talk
tags:
medicine
machine learning
artificial intelligence
Panel discussion on what is important for clinicians to know and how confident they can be when using these AI tools. Conversation with Drs. Rada Mihalcea, Max Spadafore, and Cornelius James.
Welcome to Ötleş Notes! It’s a blog by me (Erkin Ötleş).
For a little background: I am a Medical Scientist Training Program Fellow at the University of Michigan. What does that mean in English? It means I am a very silly person who decided to go to school forever in order to study medicine (MD) and engineering (PhD in industrial and operations engineering). Generally, I am fascinated by the intersection of engineering and medicine. I strongly believe that both fields have a lot to learn from one another. While working between the two presents challenges, I am genuinely grateful to learn from wonderful mentors and colleagues in both fields.
As I come across interesting topics that pertain to medicine or engineering I’ll try to share them here along with my perspective. I won’t make any guarantees regarding posting frequency or topics. However, I will to make every effort to cite original sources and be as factual as possible.
Ultimately this is a project for myself: 1) to help strengthen my written communication skills and 2) allow me to explore a broader space of ideas. If you happen to get something out of it too in the meantime that’s a wonderful byproduct.
If you have ideas about my ideas feel free to reach out to me on twitter (@eotles) or write me an email.
Cheers,
Erkin Go ÖN Home
————————
File: 2022-01-10-solving-wordle.md
Creation Date: “Sat, 27 Jul 2024 12:20:28 +0000”
—
title: “Solving Wordle”
categories:
Blog
tags:
wordle
decision science
operations research
optimization
games
artificial intelligence
machine learning
Let’s talk about Wordle. [1] You, like me, might have been drawn into this game recently, courtesy of those yellow and green squares on twitter. The rules are simple, you get 6 attempts to guess the 5 letter word. After every attempt you get feedback in the form of the colored squares around your letters. Grey means this character isn’t used at all. Yellow means that the character is used, but in a different position. Finally, green means you nailed the character to (one of) the right position(s). Here’s an example of a played game:
A valiant wordle attempt by J.B. Cheadle (January 10th 2022)
It’s pretty fun to play, although wracking your brain for 5 letter words can be annoying, especially since you are not allowed to guess words that aren’t real words (e.g., you can’t use AEIOU). Once I got the hang of the game’s mechanics my natural inclination was to not enjoy the once daily word guessing diversion, but was to find a way to “solve wordle”.
Now, what does it mean to “solve wordle”? Maybe you would like to start with a really good guess? Maybe you would like to guarantee that you win the game (i.e., guess the right word by your sixth try)? Or perhaps, you’d like to win the game and get the most amount of greens or yellow on the way? “Solving” is a subjective and probably depends on your preferences.
Due to this subjectivity I think there’s couple valid ways to tackle wordle. If you have a strong preference for one type of solution you might be able to express that directly and then solve the game in order to get the optimal way to play. I’m going to try to avoid the O-word because: 1) I don’t know what you’d like to optimize for and 2) these approaches below don’t solve for the true optimal solution (they are heuristics).
The solution strategies I’ve explored thus far can be broken down into two major categories. The first set of strategies are trying to find really good first words to start with (First Word) and the second set are finding strategies that can be used to pick good words throughout the course of the game in response to responses received from guesses (Gameplay).
Let’s start with the First Words strategies: there are two first word strategies that can be employed based on how you’d like to start your game.
First Word - Common Characters: ideal if you’d like to start your game using words that have the most common characters with all the solution words. Think of this as trying to maximize the number of yellow characters that you get on the first try.
First Word - Right Character in Right Position: ideal if you’d like to start the game using words that have the highest likelihood of having the right characters in the right position. This would yield the most number of green characters.
Rank
Solution Words
Usable Words
1st
later, alter, alert
oater, orate, roate
2nd
sonic, scion
lysin
2nd
pudgy
chump :)
First Word - Right Character in Right Position: ideal if you’d like to start the game using words that have the highest likelihood of having the right characters in the right position. This would yield the most number of green characters.
Rank
Solution (& Usable) Words
1st
slate
2nd
crony
2nd
build
Note on solution word vs. usable words. Wordle has two sets of words, solution words and other words. Other words are never the correct answer but can be used as a guess. There’s a chance that other words can be used to get a lot of yellows, despite never being the correct answer. So I created a list of usable words that combined the solution words and the other words. Notice that the First Word - Common Characters strategy has two lists. That’s because there are other words like “oater” that are more likely to produce yellows than the best solution word “later”. This isn’t the case for the First Word - Right Character in Right Position, as it produces the same results for both sets of words.
You might also observe that there are several sets of words in terms of 1st, 2nd, and 3rd. If you wanted you could use these strategies over several rounds to build up your knowledge. However, these strategies don’t take into account the feedback that you get from the game. So there may be better ways to play the game that take into account what kind of results you get after you put in a guess.
These strategies are the Gameplay strategies. I’ll present two potential approaches that use knowledge as it is collected.
Gameplay - Refine List + Common Characters: this one works by sifting through the remaining words that are feasible (e.g., don’t use grey characters and have green characters in the right spot) and then uses the Common Characters approach to rank the potential word choices that remain.
Gameplay - Reinforcement Learning: this one works by learning what is the best word to guess given what you have guessed in the past. [2] It does this learning by playing the Wordle many times (e.g., millions) and then collecting a reward based on how it does (+1 point for winning and 0 points for losing). Over repeated plays of the game we can learn what guesses might lead to winning based on the current state of the game.
Here is an example of the Gameplay - Refine List + Common Characters strategy in action based on the Wordle from January 10th 2022.
Guess #
Green Characters
Grey Characters
Guess
Result
1
*****
alert
2
**\er*
a, l, t
fiery
3
**\ery*
a, f, i, l, t
query
Here you can see that after every guess we get to update the green characters and the grey characters that we know about. For example after round 1, we know that the word must be **er* (where * represent wildcards) and must not contain the characters: a, l (el) or t. I use regular expressions to search through the list of words, the search expression is really simple, it just replaces * in the green character string with tokens for the remaining viable characters (the set of alphabet characters minus the grey characters).
The reinforcement learning based approach would operate in a similar manner for a user. However, the mechanics under the hood are a bit more complicated. If you are interested in how it (or any of the other strategies) work please see the appendix.
As I mentioned above, solving wordle is subjective. You might not like my approaches or might think there are ways for them to be improved. Luckily I’m not the only one thinking about this problem. [3, 4]
This contains some technical descriptions of the approaches described above.
First Word - Common Characters
This one is pretty simple. I am essentially trying to find the word that has the most unique characters in common with other words (this is a yellow match).
In order to do this I reduce words down to character strings which are just lists of unique characters that the words are made up of. So for an example, the word “savvy” becomes the string list: a,s,v,y. We then use the chapter strings to count the number of words represented by a character. So using the character string from above the characters a, s, v, and y would all have their counts incremented by 1. These counts represent the number of words covered by a character (word coverage).
We then search through all words and calculate their total word coverage. This is done by summing up the counts for every character in the word. We then select the word with the highest amount of other word coverage. In order to find words to be used in subsequent rounds we can remove the characters already covered by previously selected words and repeats the previous step.
This one is a pretty straightforward extension of the First Word - Common Characters approach that has an added constraint, which is position must be tracked along with the characters.
To do this we count a character-position tuples. For every word we loop through the characters and their positions. We keep track of the number of times a character-position is observed. For example, the world “savvy” would increment the counts for the following character-portion tuples: (s, 1), (a, 2), (v, 3), (v, 4), (y, 5). These counts represent the number of words covered by a character-tuple (word coverage).
We then loop through every word and calculate their total word coverage. This is done by breaking the word into character-position tuples and summing up the counts of the observed character-positions.
Both the First Word strategies can be converted from counts to probabilities. I haven’t done this yet, but maybe I’ll update this post in the future to have that information.
The Gameplay strategies are a little more complicated than the First Word strategies because they need to be able to incorporate the state of the game into the suggestion for the next move.
Gameplay - Refine List + Common Characters
This approach is reminds me of an AI TA I had. He would always say “AI is just search”. Which is true. This approach is pretty much searching over the word list with some filtering and using some distributional knowledge. It was surprised at how easily it came together and how effective it is. As a side note, it was probably the easiest application of regex that I’ve had in a while.
There are three components to this approach:
Generate Regex: build the search filter
Get possible solutions: apply filter to the word list
Rank order solutions: apply common character counting on the filtered word list
I will briefly detail some of the intricacies of these components.
Generate Regex: the users need to provide 3 things before a guess 1) a string with the green characters positioned correctly and wildcards (*) elsewhere, 2) a list of the yellow characters found thus far, and finally 3) a list of the gray characters. Using this information we build a regular expression that describes the structure of the word we are looking for. For example let’s say we had **ery as green letters and every character other than q and u were greyed out then we would have a regex search pattern as follows: [qu][qu]ery.
Get possible solutions: after building the regex search string we can loop through the list of solution words and filter all the words that don’t meet the regex search pattern. We can additionally remove any words that do not use characters from the yellow characters list. Finally, we then Rank Order Solutions by finding each words coverage using the approach described in Common Characters above. This produces a list of words ranked by their likelihood of producing yellow characters on the remaining possible words.
This approach is based on tabular Q-learning. [2, 5] Its a little bit complicated and I’m unsure the training procedure produced ideal results. But I’ll provide a brief overview.
Reinforcement learning seeks to learn the right action to take in a given state. [6] You can use it to learn how to play games if you can formulate that game as a series of states (e.g., representing a board position) and actions (potential moves to take). [5] In order to convert tackle the wordle task with RL we need a way to represent the guesses that we’ve already done (state) and the next guess we should make (action).
The actions are pretty obvious, have one action for each potential solution word we can guess. There’s about 2,000 of these.
The states are where things get hairy. If you wanted to encode all the information that the keyboard contains you would need at least 4^26 states. This is because there are 4 states a character can take {black/un-guessed, yellow, green, grey} each character can be in anyone of these states. This is problematic - way too big! Additionally, this doesn’t encode the guesses we have tied. What I eventually settled on was a state representation that combined the last guessed word along with the results (the colors) for each character. This is a much more manageable 2,000 x 4^5.
I then coded up the wordle game and used tabular Q-learning to learn the value of state action pairs. This was done through rewarding games that resulted in a win with a 1 and losses getting a 0.
I think this also might be solvable using dynamic programming as we know the winning states. These are terminal and then I think you can work backwards to assign values to the intermediary states. It’s been almost a decade since I took my dynamic programming class, so I need a bit of a refresher before I dive into it.
As you can see, there are a lot of interesting questions that arise from formulating this task as an RL problem. I will probably come back to this and explore it further in the future.
Bibliography
Wordle - A daily word game. 2022; Available from: https://www.powerlanguage.co.uk/wordle/.
Q-Learning - An introduction through a simple table based implementation with learning rate, discount factor and exploration - gotensor. 2019.
Solve Wordle. 2022; Available from: https://www.solvewordle.com/.
Glaiel, T., The mathematically optimal first guess in Wordle. 2022.
Friedrich, C., Part 3 - Tabular Q Learning, a Tic Tac Toe player that gets better and better. 2018.
Sutton, R.S. and A.G. Barto, Reinforcement learning : an introduction. Adaptive computation and machine learning. 1998, Cambridge, Mass.: MIT Press. xviii, 322 p.
Wordle Solver by eotles
------------------------
File: 2022-01-14-mouse-cursor-flip.md
Creation Date: "Sat, 27 Jul 2024 12:20:28 +0000"
---
title: "#@!% Flipping Cursor!"
categories:
- Blog
tags:
- UI/UX
- human factors engineering
- healthcare IT
- Microsoft Word
- mouse cursor
---
ecently I came across some interesting behavior in Microsoft Word. While scrolling through a document I noticed that my pointer had flipped. Instead of seeing the classic arrow pointer (pointing to the upper-left) the pointer had flipped horizontally (arrow pointing to the upper-right). [1] Jiggling the pointer on and off the Word application caused the arrow pointer to flip back-and-forth. A video highlighting this behavior is embedded below.
The pointer starts out as a normal arrow pointer then changes to a horizontal I beam pointer once the Word application is brought into focus by clicking. As the pointer travels left the pointer switches to a flipped arrow pointer. Traveling to the right we see the horizontal I beam pointer and eventually the normally expected classic arrow pointer. What the #$@!%?
It took me a while to figure this out, because googling “flipped reversed pointer cursor” primarily gives you stuff pertaining to mouse scrolling direction. But I eventually happened across a helpful StackExchange discussion. [2] Apparently, this is meant to be a useful feature for users. If you click when the pointer is in the flipped configuration Word will highlight the corresponding line of text, see example video below:
Once you know about this you might consider it helpful. But really?! It is a buried feature that leads to two outcomes: 1) it doesn’t get noticed by the majority of users or 2) when it does get noticed it causes confusion (🙋🏾♂️). Apparently, other MS Office applications do similar things when the pointer goes leftward. [2] However, the Microsoft pointer UI documentation has no mention of why or when a flipped arrow pointer is supposed to be employed. [3]
Maybe I’m totally off-base. Maybe the flipped arrow pointer in MS Office applications leads to features that are loved by the masses. Maybe I have just missed this particular train? Probably not. I have a tendency to agree with the JohnGB on StackExchange that: “Consistency matters in UX, even when it is in things that most people will not be able to consciously notice.”
I think this is a good parting thought, it is especially salient for those of us that work in healthcare IT. The mental workload in healthcare is taxing, so software user experiences should be as simple as possible. There’s no reason to confuse your users by adding complexity and breaking your own design rules, especially if you aren’t providing substantial value.
Erkin
[Go ÖN Home](../../index.md)
Note: the discrepancy in verbiage between the title and the text. Mouse cursor and pointer seem to be interchangeable when referring to the “pointy thing”. [4] I use pointer through the text as that’s what Apple’s human interface guidelines call it. [1] But the codebase refers to NSCursor, so 🤷🏾♂️.
Note 2: below are the versions of the software I was using.
MacOS: 12.0.1 (21A559)
Word 16.56 (21121100)
Pages: 11.2 (7032.0.145)
Note 3: it is annoying that you can’t copy the version number from the About Word window of Microsoft Word.
## Bibliography
1. Apple. Human Interface Guidelines: Mouse and Trackpad. 2022; Available from: https://developer.apple.com/design/human-interface-guidelines/macos/user-interaction/mouse-and-trackpad/.
2. @StackUX. When to use reversed/mirror arrow cursor? 2022; Available from: https://ux.stackexchange.com/questions/35435/when-to-use-reversed-mirror-arrow-cursor.
3. hickeys. Mouse and Pointers - Win32 apps. 2022; Available from: https://docs.microsoft.com/en-us/windows/win32/uxguide/inter-mouse.
4. Cursor (user interface) - Wikipedia. 2022; Available from: https://en.wikipedia.org/wiki/Cursor_(user_interface).
------------------------
File: 2022-01-17-plutonium-pacemakers.md
Creation Date: "Sat, 27 Jul 2024 12:20:28 +0000"
---
title: "Plutonium Pacemakers"
categories:
- Blog
tags:
- medicine
- heart
- cardiac pacemaker
- nuclear power
- pacemaker
- engineering
- biomedical devices
---
This is a reformatted version of a [twitter thread I had put together nearly a year ago](https://twitter.com/eotles/status/1370206446881701891?s=20). In a former life I worked on designing the manufacturing system for cardiac pacemakers. I had done a bit of research on pacemakers at the time, but I had never come across the fact that some early pacemakers were designed and built with plutonium power sources.
Begin reformatted thread:
Fell down a history hole and came across the fact that we used to implant plutonium (!) powered cardiac pacemakers ❤️⚡️☢️
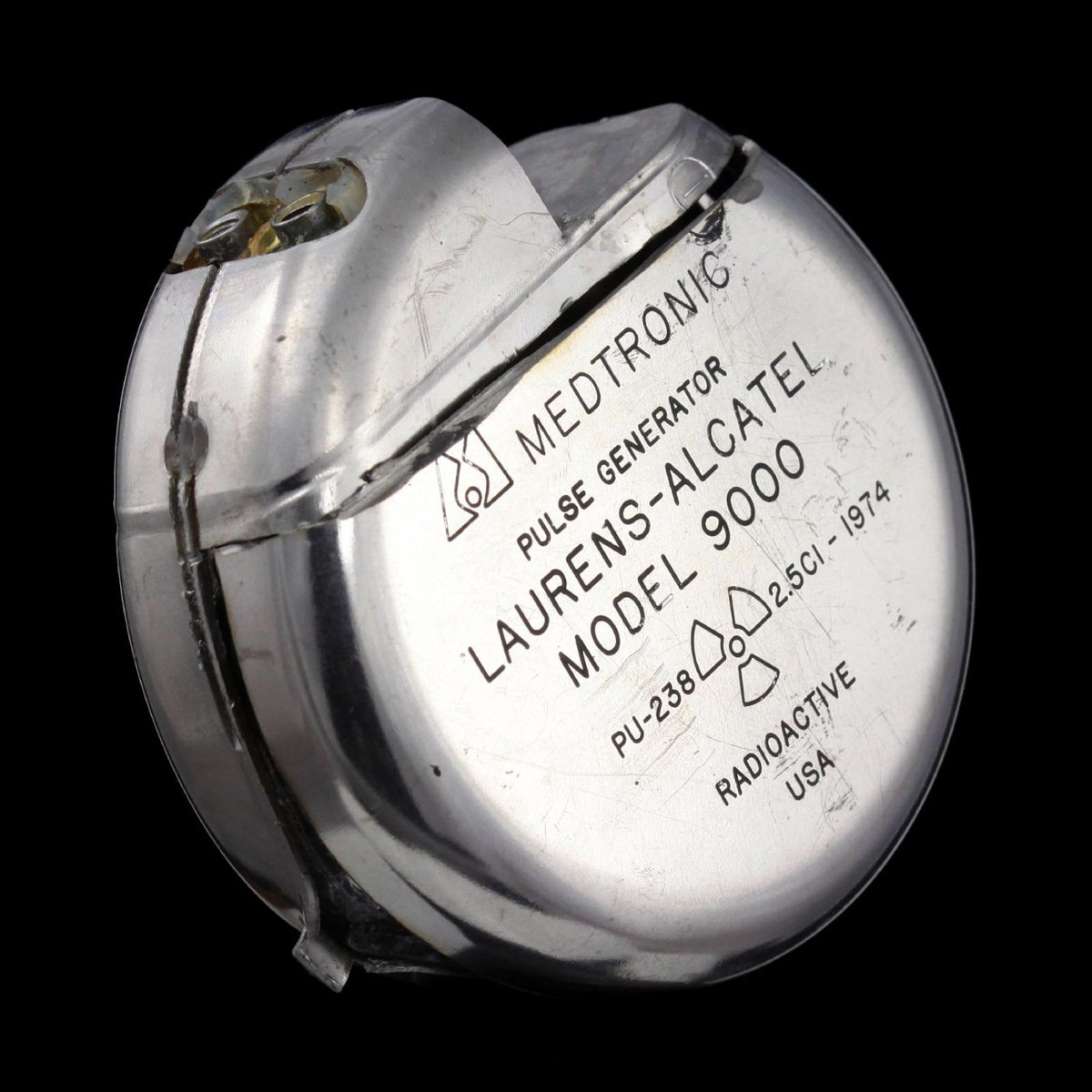
Below is a cutaway schematic - they used the heat generated from radioactive decay to generate electricity using thermocouples [1]
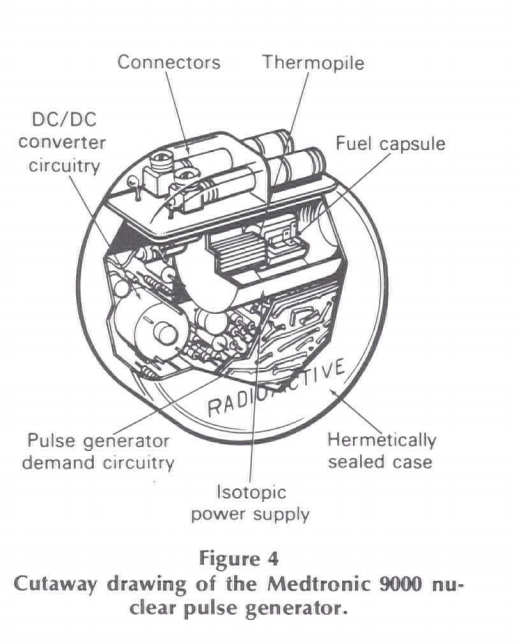
Why nuclear power? In the early days if you wanted to pace a patient for a long time (i.e. a pediatric patient) you would need to replace the pacing device a lot because the batteries would die 🔋😧 [2]
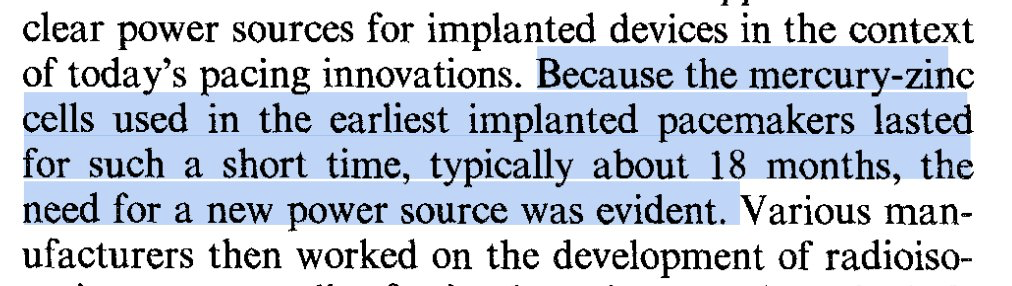
In order to sell these in the US you needed sign-off from both @US_FDA and the @NRCgov (nuclear regulatory commission). of course @Medtronic made one, but apparently a bunch other folks got in the game as well - including monsanto! [3]
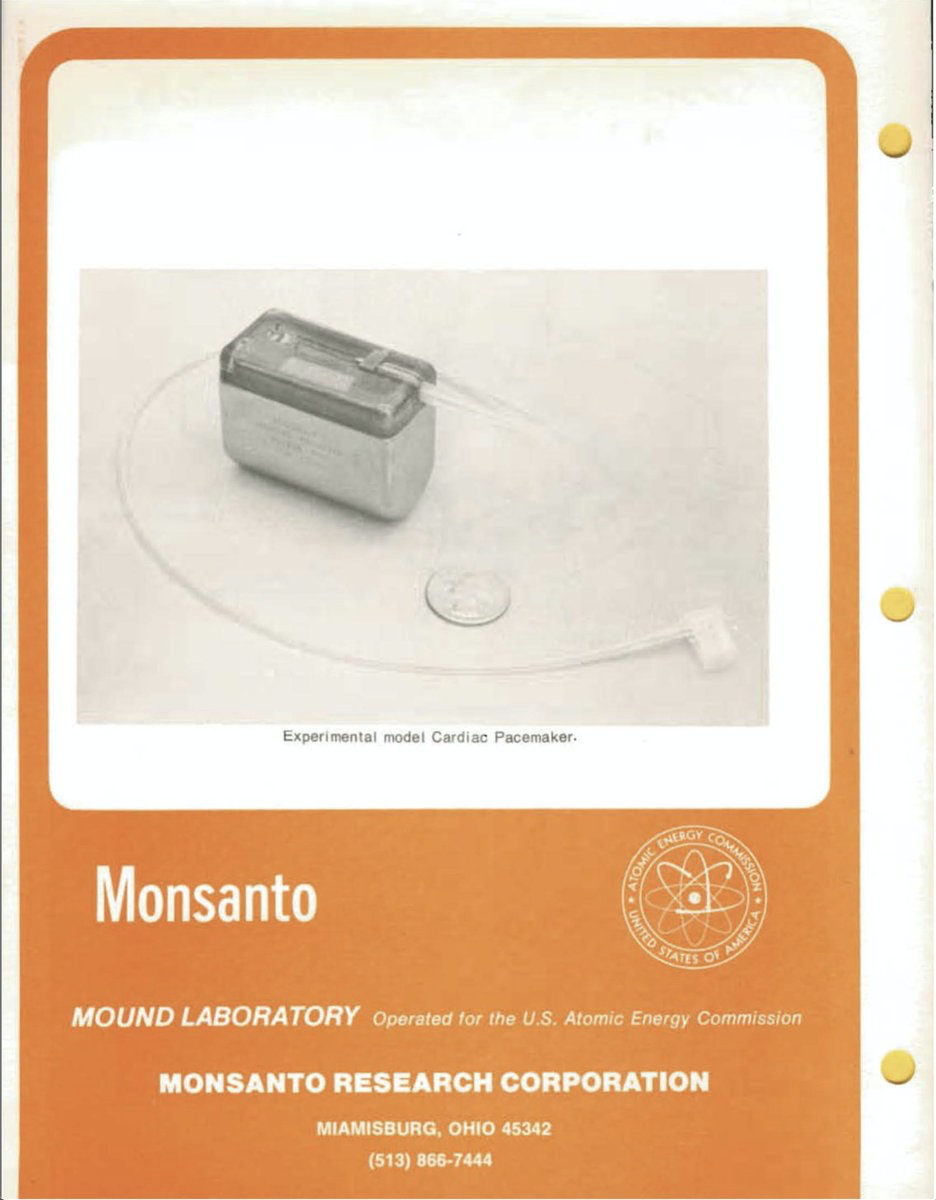
As weird as it sounds people were 𝕚𝕟𝕥𝕠 the concept of having plutonium powered pacemakers at the time. [2]
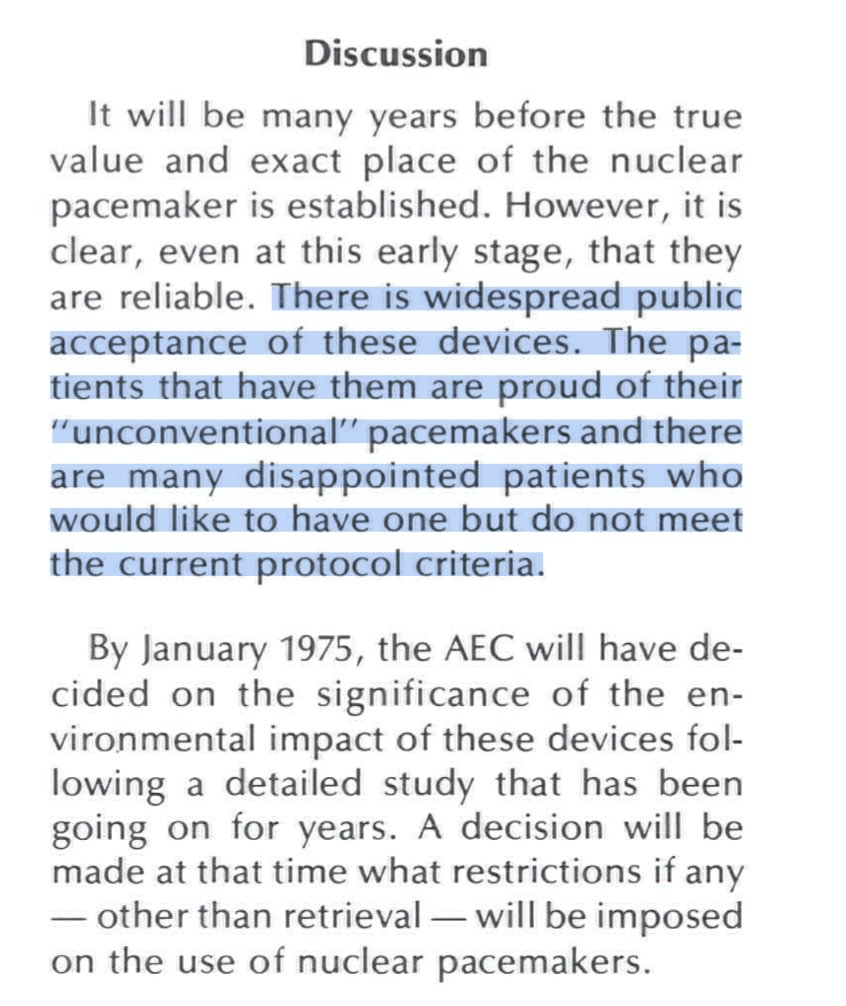
Radiation exposure was a concern, although theoretically the devices were well shielded and risk would be minimal. theory was borne out in practice - after years of study it turned out that patients with these pacemakers did NOT have higher rates of cancer. [4]
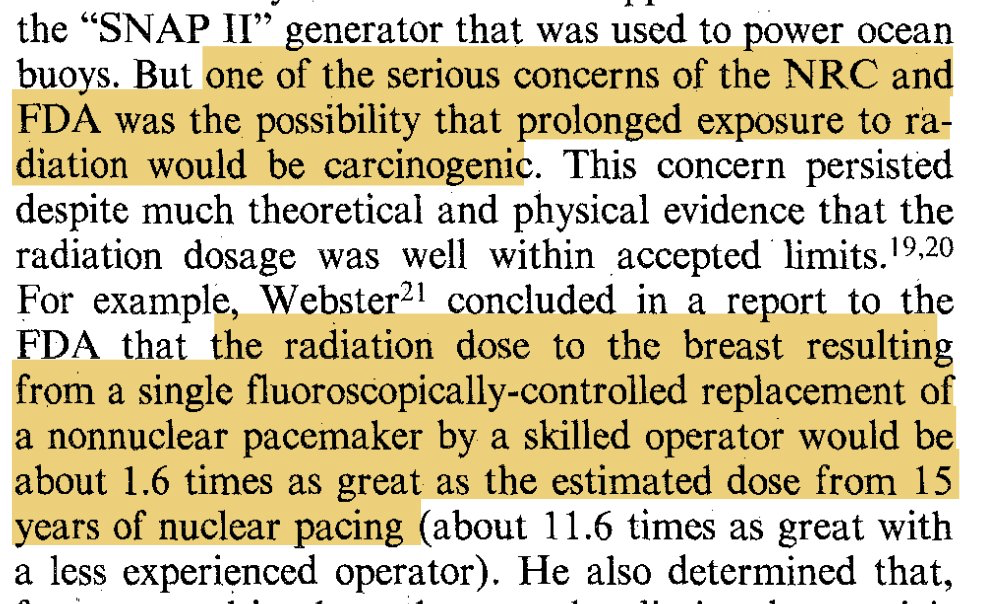
Thousands of these pacemakers were implanted in the 70s and it turns out that they lasted for a very long time. in 2007 a case report was written about a pacemaker that was still firing since its implantation in 1973! 😧 [5]
This crazy longevity wasn't necessarily a great thing - replacements = better features (i.e. interrogation and programming). plus end-of-life disposal issues made plutonium pacemakers a poor choice once better batteries came along.
On one hand the logic behind why you would design and implant these pacemakers makes total sense and on the other its totally wild because of the current stigma associated with everything nuclear.
Erkin
[Go ÖN Home](../../index.md)
## Bibliography
1. Radioisotope thermoelectric generator - Wikipedia. 2022; Available from: https://en.wikipedia.org/wiki/Radioisotope_thermoelectric_generator.
2. Smyth, N.P., T. Hernandez, and A. Johnson, Clinical experience with radioisotopic powered cardiac pacemakers. Henry Ford Hospital Medical Journal, 1974. 22(3): p. 113-116.
3. Wayback Machine - Cardiac Pacemaker. 2022; Available from: https://web.archive.org/web/20160816084535/https://dl.dropboxusercontent.com/u/77675434/Heat%20Source%20Datasheets/CARDIAC%20PACEMAKER.pdf.
4. Parsonnet, V., A.D. Berstein, and G.Y. Perry, The nuclear pacemaker: Is renewed interest warranted? The American Journal of Cardiology, 1990. 66(10): p. 837-842.
5. Parsonnet, V., A lifetime pacemaker revisited. New England Journal of Medicine, 2007. 357(25): p. 2638-2639.
------------------------
File: 2022-01-24-looking-for-data.md
Creation Date: "Sat, 27 Jul 2024 12:20:28 +0000"
---
title: "Looking for Data"
categories:
- Blog
tags:
- healthcare
- medicine
- data
- data science
- machine learning
- operations research
- nurse call light system
---
One of the nice things about being an MD-PhD student at a research institution with a large academic medical center is that you tend to have a lot of support when it comes to working on your biomedical research questions. Despite institutional support, data can be a challenge and finding the right data for your question depends a lot on your connections with the myriad various data systems and data-gate-keepers that exist in your academic environment. Having done this data sleuthing for a decade plus I have bit of experience in ferreting out interesting sources of healthcare data.
One of my favorite data finds of all time was from a project I led when I was just starting out as quality improvement engineering for a hospital. I had been tasked with redesigning the inpatient rooms of the academic medical center I was working for. A significant portion of the project was blue-sky/brainstorming type engineering. But there was a portion of the project that involved troubleshooting the layout of an existing unit that had been receiving lots of complaints from nurses and CRNAs.
In order to benchmark the current unit and to help inform planned changes we needed to understand the flow of work done by the nursing staff. Our typical approach for this type of data collection was to collect spaghetti diagrams. A spaghetti diagram is a simple, but effective, chart that maps the travel path of a person or an object over a given duration. [1] When complete the travel path looks like a plate of spaghetti has been spilled on a floor plan. Making spaghetti diagrams is a time consuming process, as you need an observer to track the target person (in our case nurses or CRNAs) for long periods of time. After drawing the short-straw I found myself on the night shift shadowing the superb night team of the unit.
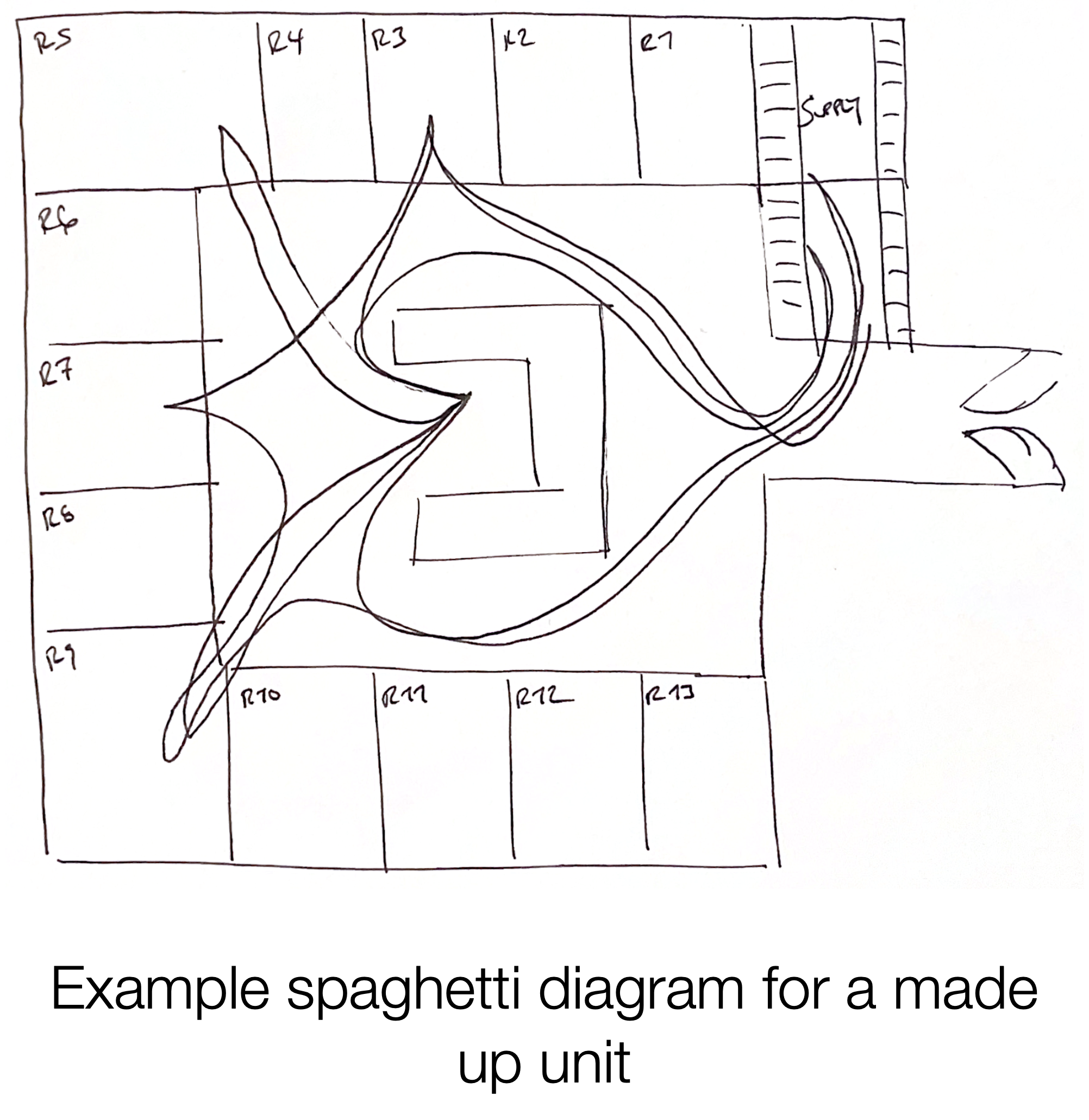
Halfway through my night shift I started wondering if there was a better way to be collecting this information. What we really were after was how often do the nurses need to leave a patient’s room because they are missing supplies and how long does this take them? Was there another way to collect this data without having to sacrifice sleep and (more importantly) not bothering nurses and patients?
I noticed that every time the nurse I shadowed entered a patient’s room there was a light above the patient’s room that lit up. When they left the room the light went dark. I inquired about the lights and learned from the nurse that I was shadowing that they were part of the nurse call light system, which is a like a souped up airplane flight attendant call light system. [2] In addition to indicating if a patient had a request it had the capability to show the presence of a nurse in a room. Additionally, I learned that this system was all wired up such that the unit coordinator (front desk of the unit) was the person that received the patient request calls and they also had a light board representing the status of the whole unit so that they could coordinate requests with nursing staff.
So, what initially seemed like a simple light switch turned out to be fairly complicated system. I figured that there must be a computer system facilitating this complexity. And if there was a computer involved in exchanging data then there was a chance it might also be storing data. And if I could get access to this data I might be able to answer my unit redesign questions without having to pull too many more night shifts. And I might be able to avoid bothering nurses and patients.
After leaving my shift with a stack of scribbles I emailed my supervisor inquiring about the call light system. She did a bit of hunting and found the people responsible for the call light system. After meeting with them we found out that the system did store data and that we could use it for our project, if we agreed to certain (very reasonable) terms of use.
We got the data. It was in the form of logs recording every timestamp a staff ID badge entered a different room. I whipped up a java program to analyze the amount of time nursing staff were in a patient’s room and the number of times they had to bounce between patient rooms and the supply rooms. It turns out the unit we were studying did have a problem with staff needing to leave the room frequently and rooms in that unit were slotted to be remodeled with more storage.
My big takeaway from this experience is that there’s alway a chance that there’s a good dataset that exists, but you won’t get access to it if you don’t do the work to look for it. And sometimes doing that work is easier than doing the work to collect your own data. :)
Erkin
[Go ÖN Home](../../index.md)
P.S. I started this post with some notes on gaining access to the typical datastore in academic medical settings. I have some additional thought about these data systems (e.g., discussing how they are typically structured and some of the things to look out for when using them) if you’re interested let me know and I’ll prioritize writing that up for a future post.
## Acknowledgements
I’d like to thank [Zoey Chopra](https://www.linkedin.com/in/zoeychopra/) for catching a redundant paragraph.
## Bibliography
1. What is a Spaghetti Diagram, Chart or Map? | ASQ. 2022; Available from: https://asq.org/quality-resources/spaghetti-diagram.
2. NaviCare⢠Nurse Call | hill-rom.com. 2022; Available from: https://www.hill-rom.com/international/Products/Products-by-Category/clinical-workflow-solutions/NaviCare-Nurse-Call/.
------------------------
File: 2022-01-29-b52-health-IT.md
Creation Date: "Sat, 27 Jul 2024 12:20:28 +0000"
---
title: "What Can Healthcare IT Learn from the B-52?"
categories:
- Blog
tags:
- healthcare IT
- maintenance
- upgrades
- aerospace engineering
- technical debt
- total cost of ownership
---
A lot of aviation videos show up on my YouTube feed (thank you, [DarkAero](https://www.youtube.com/c/darkaeroinc) team). A video that popped up recently was about the Boeing B-52 Stratofortress (B-52) engine retrofit project. According to wikipedia the B-52 is a “long-range, subsonic, jet-powered strategic bomber” that has been used by the US Air Force since 1955. [1] Despite being designed and built 6 decades ago, the US Air Force still uses these planes and plans on using them well into the future. This desire to keep using them into the future is where things get interesting and we in healthcare IT can learn some lessons.
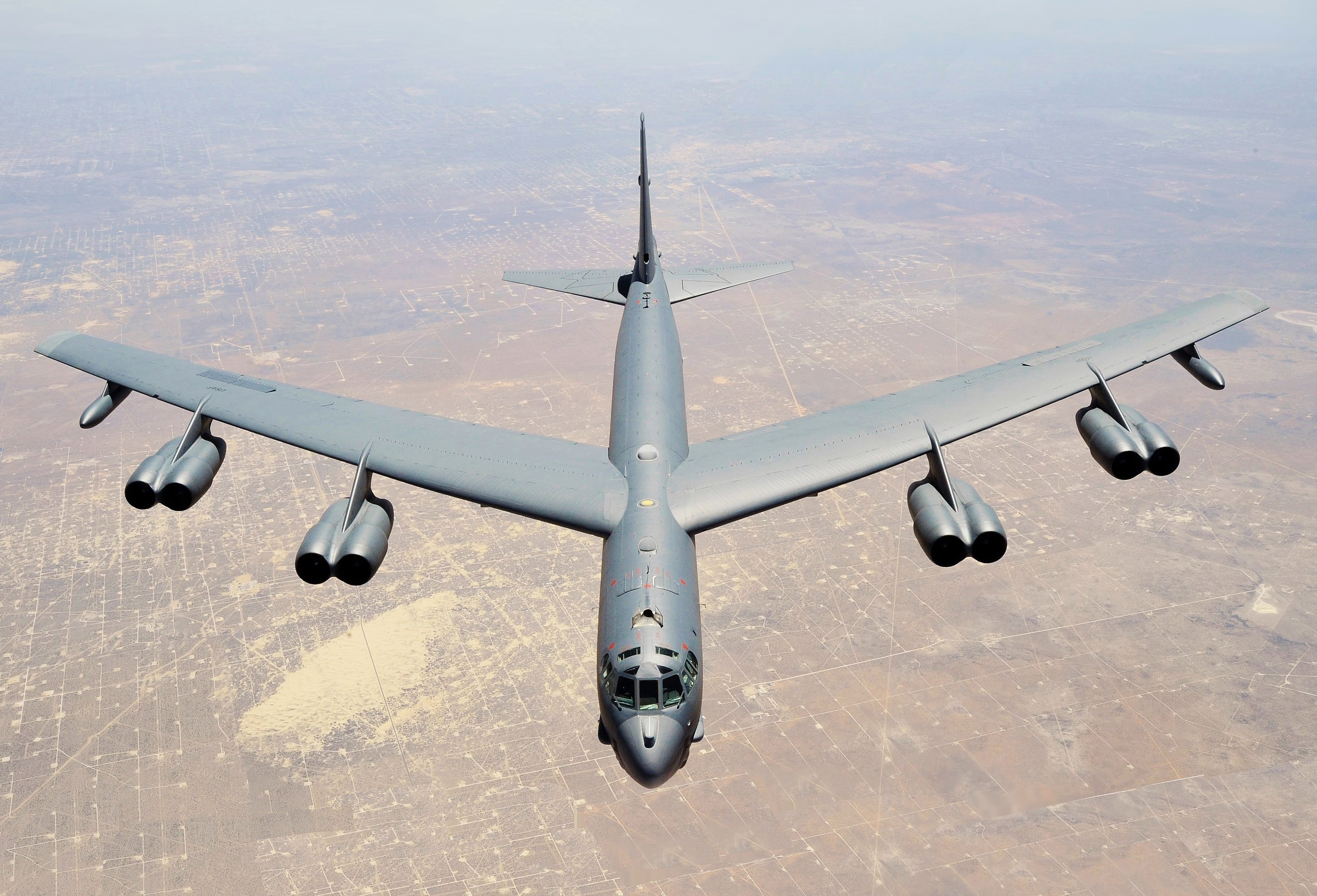
As an aside, my personal belief is machines like this are pretty odious. I like machines, I like making physical things, and I like planes. But when the thing in question is expressly designed to kill people and destroy property, I start to have some problems. Obviously there’s a reason why these exist (and why they’ve been used) but I find their existence troubling and I wish we lived in a world where these types of machines did not have to exist.
The upgrading of these planes is covered well by wikipedia, an Air Force Magazine article, and the original YouTube video that sparked my interest in the topic. [1-3] Basically, the last B-52 rolled off the assembly line in 1962 and the Air Force has been refurbishing the original engines as time has gone on. In order to keep the planes flying into the 2040s the US government has decided to order new engines for the existing planes. Note an emerging connection, both the US government and US healthcare organizations are loathe to let old technology die. We gotta squeeze all the usable life out of those faxing systems…
New engines old plane, makes sense right? Sure, but take another glance at the B-52 (image above). Look at how many engines there are. Four pairs of small jet engines, for a total of 8 engines! Seems like we have an opportunity to cut down on the number of engines, right? Two turbofan jet engines is the standard for most modern commercial aircraft being delivered by Boeing or Airbus these days. Even if we didn’t go down to two we could go down to four easily. No need to change the number of mounting points! This is very logical, but it’s not truly feasible. Why? Because of design decisions made 69 years ago.
This underscores a concept that is not discussed widely enough in healthcare IT circles:
> Your choices for tomorrow are ultimately constrained by what you designed yesterday.
The jet engine technology of the 1950s ultimately informed how the rest of the B-52 was designed. The references go into more detail, but if you were to re-engine the B-52 with a smaller number of more powerful engines you would have to totally redesign other parts of the plane. For example the rudder, wings, and control systems would have to totally be redesigned. Doing that might mean that you’d have to rethink the fuselage as well. You would be better off designing a new airplane from the ground up. So the choice becomes maintain with significant constraints or totally redo.
When thinking about the health IT landscape we can see this concept everywhere. Why do we still put up with aging faxing servers and paging systems that are down more often than not? Because we built a system around them and the costs associated with their wholesale replacement are not tenable. Healthcare IT budgets are not infinite, so more often than not we have to focus on how to keep things going by repeatedly doing smaller upgrades. The best we can do is to try to strike a balance between current capabilities and future-proofing.
Even though the B-52 engine retrofit project is significantly constrained, the fact that we are still able to use it at all and will be able to keep it flying till 2040 is a testament to the prowess of the original engineers. And all the engineers who have worked on it since. There is an aspect to this longevity that is inspiring. However, it is important to ask: would it have been better to do a clean sheet design and pay-off the accrued technical debt? [4]
This is a question that can be asked of healthcare IT as easily as it can be asked of the US military. Heck, over half of all patient in the US have their electronic health records coded up in a programming language that was originally released in 1966. [5, 6] Both healthcare IT and the US military are ponderous creatures that generally ascribe to “if it ain’t totally broke don’t fix it”. There’s a lot more to discuss on this topic. It closely relates to the concept of total cost of ownership (might dive into in the future). But its important to recognize how the decisions we make today will impact the decisions we can make in the future.
Youtube video embedded below:
Erkin
[Go ÖN Home](../../index.md)
## Bibliography
1. Boeing B-52 Stratofortress - Wikipedia. 2022; Available from: https://en.wikipedia.org/wiki/Boeing_B-52_Stratofortress.
2. The B-52 is Getting New Engines... Why Does it Still Need 8 of Them?
3. Tirpak, J.A. Re-Engining the B-52. 2019; Available from: https://www.airforcemag.com/article/re-engining-the-b-52/.
4. Technical debt - Wikipedia. 2022; Available from: https://en.wikipedia.org/wiki/Technical_debt.
5. MUMPS - Wikipedia. 2022; Available from: https://en.wikipedia.org/wiki/MUMPS.
6. JEFF GLAZE jglaze@madison.com, -.-. Epic Systems draws on literature greats for its next expansion. 2022.
------------------------
File: 2022-02-01-Development-and-Validation-of-Models-to-Predict-Pathological-Outcomes-of-Radical-Prostatectomy-in-Regional-and-National-Cohorts.md
Creation Date: "Sat, 27 Jul 2024 12:20:28 +0000"
---
title: "Development and Validation of Models to Predict Pathological Outcomes of Radical Prostatectomy in Regional and National Cohorts"
categories:
- Blog
- Research
tags:
- Blog
- Research
- urology
- radical prostatectomy
- prostate cancer
- medicine
- healthcare
- artificial intelligence
- machine learning
---
The Journal of Urology article, read [here](https://doi.org/10.1097/JU.0000000000002230).
[Download paper.](https://eotles.com/assets/papers/development_and_validation_of_models_to_predict_pathological_outcomes_of_radical_prostatectomy.pdf)
## Abstract
### Purpose
Prediction models are recommended by national guidelines to support clinical decision making in prostate cancer. Existing models to predict pathological outcomes of radical prostatectomy (RP)—the Memorial Sloan Kettering (MSK) models, Partin tables, and the Briganti nomogram—have been developed using data from tertiary care centers and may not generalize well to other settings.
### Materials and Methods
Data from a regional cohort (Michigan Urological Surgery Improvement Collaborative [MUSIC]) were used to develop models to predict extraprostatic extension (EPE), seminal vesicle invasion (SVI), lymph node invasion (LNI), and nonorgan-confined disease (NOCD) in patients undergoing RP. The MUSIC models were compared against the MSK models, Partin tables, and Briganti nomogram (for LNI) using data from a national cohort (Surveillance, Epidemiology, and End Results [SEER] registry).
### Results
We identified 7,491 eligible patients in the SEER registry. The MUSIC model had good discrimination (SEER AUC EPE: 0.77; SVI: 0.80; LNI: 0.83; NOCD: 0.77) and was well calibrated. While the MSK models had similar discrimination to the MUSIC models (SEER AUC EPE: 0.76; SVI: 0.80; LNI: 0.84; NOCD: 0.76), they overestimated the risk of EPE, LNI, and NOCD. The Partin tables had inferior discrimination (SEER AUC EPE: 0.67; SVI: 0.76; LNI: 0.69; NOCD: 0.72) as compared to other models. The Briganti LNI nomogram had an AUC of 0.81 in SEER but overestimated the risk.
### Conclusions
New models developed using the MUSIC registry outperformed existing models and should be considered as potential replacements for the prediction of pathological outcomes in prostate cancer.
------------------------
File: 2022-02-06-ehr-front-ends.md
Creation Date: "Sat, 27 Jul 2024 12:20:28 +0000"
---
title: "The Complicated Way You See Patient Data: A Discussion on EHR Front-Ends for Doctors"
categories:
- Blog
tags:
- healthcare IT
- electronic health records
- computer science
- software engineering
- software architecture
- clinical informatics
- tech support
---
I have a love-hate relationship with electronic health records (EHRs). This relationship first started in the early 2000s at a high school sports physical and has significantly outlasted my high school soccer career. Eventually the relationship turned serious and my first job out of college was for an EHR vendor. My thrilling life as a support engineer at Epic Systems Corporation was cut short by my silly decision to pursue an MD-PhD. After years of being on one side of the software and data-stack I transitioned to being a “user" for the first time. While not totally naive to all of the issues surrounding modern EHRs this transition was still pretty eye opening.
I believe a significant subset of these issues actually stem from a general lack of communication between the engineering community making these tools and the medical community using them. One of my goals in pursuing the MD-PhD was to hopefully help bridge this gap a little bit. As such, I’m usually game to play tech support on the wards and I like explaining how the software we use works (or doesn’t). I also like translating what we do in medicine to the engineers that will listen. Basically I’ll talk to any crowd that will listen (maybe this is why I went into academia 🤔).
The complicated way we see patient data.
This post is inspired by a tech support call I fielded from Jacob, one of my med school classmates. Jacob was about to take an overnight call shift and his computer was displaying the EHR in a manner that made the font so small it wasn’t readable. I walked through some potential settings in the EHR that could be affecting what he was seeing, but everything we tried came up short. Eventually Jacob texted his co-resident and they told him to try modifying a Citrix Receiver setting, which worked. My singular focus on the complexity inside of the EHR instead of the complexity AROUND the EHR led to my tech-support failure. The complexity around the EHR will be the focus of this blog post.
Concurrently serving an EHR to thousands of physicians, nurses, and allied health professionals across health systems is a big task. This task, like most other software tasks that involve interacting with users, is broken into two big components, with a front-end and a back-end. [1] This is an over simplification, but the front-end is everything that a user interacts with and the back-end is all the other stuff that needs to exist in order to store and transmit data used by the front end. You’ve probably been the beneficiary of this division of labor even if you’ve never written any code. Twitter, Facebook, Youtube, and Gmail all use this approach.
Let’s take Gmail. The front-end of Gmail is all the code that needs to run on your laptop (or phone) in order for Gmail to show you your emails. The back-end of Gmail is all of the code that Google needs to run in order to store your emails, send your outgoing emails, and receive your incoming emails. In order for you to see your emails Gmail’s front-end and back-end need to communicate, they do this by passing messages back and forth. A similar setup is employed with EHRs. The front-end of the EHR is what shows you the lab values of a patient. The back-end is what ultimately stores those lab values along with notes and other data.
This separation of front-end-back-end makes engineering easier as it decouples the information presentation functions from the functions that actually run the service. This allows engineers to upgrade the look and feel of a website without having to worry about redesigning the way the site interacts with a database. Ultimately this separation enables specialization and efficiency. One set of engineers can focus on making the front-end look good and another set can focus on making the back-end run fast. As long as these engineers trust each another they work efficiently by focusing on their own domains.
The software that makes up the front-end is often known as the client. The amalgamation of everything on the back-end is often known as the sever. [2] Its a little facetious to talk about a single client and a single server, because most well-known projects might have multiple clients and many servers. However, its not too far off from the current state that most EHR users are familiar with. For this post we will keep our focus on the front-end/client side of things.
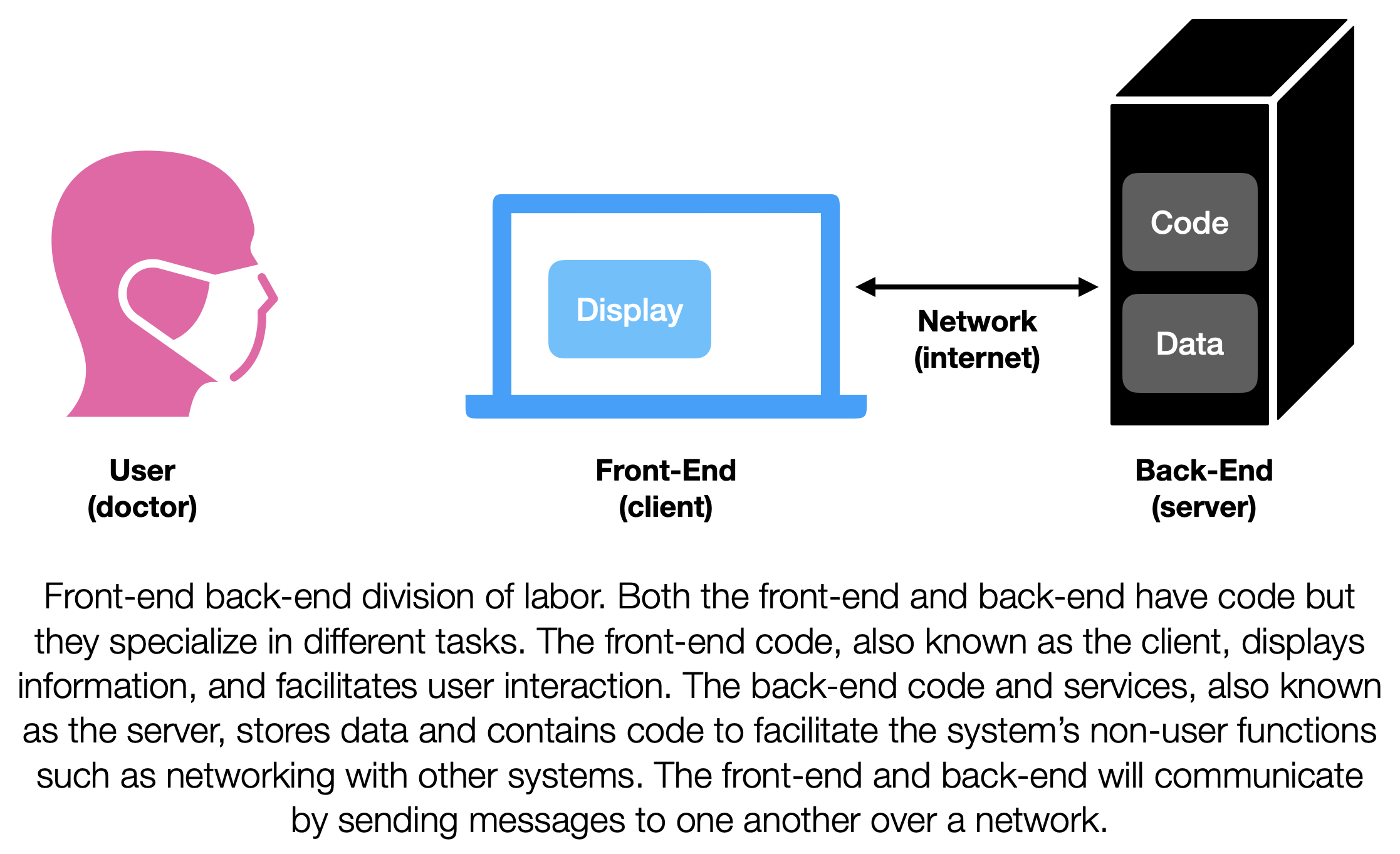
Let’s stick with Epic’s EHR system. The client most everyone is familiar with is Hyperspace, which can be found in clinics and hospitals all over the US. [3] I don’t know if there’s any data on this but I’d hazard a guess that the Hyperspace client accounts for over 95% of the total time users spend with Epic’s EHR. (That guess is based on my own usage time as a med student.) Although I mainly used Hyperspace, I would occasionally check up on my patients using Haiku or Canto. Haiku is a client designed for smartphones (there are apps for both Android and iOS) and Canto is a client designed for iPads. Additionally as a patient I use MyChart to access my own medical records. All of these clients are designed with different goals in mind and provide differing access to clinical information and workflows.
Each one of these clients needs code in order to display information and facilitate user interaction. Usually clients accomplish this by having code that runs on the machine the user is using. For example the code for Canto is downloaded on my iPad. When I click on a patient’s name on Canto code executes (that code was probably written in the Swift language). That Swift code may change what is displayed on the screen and may also send or receive messages from servers. It may do any number of additional things, but the primary user interaction and communication tasks are handled by code that is running on my iPad. This set up is pretty similar for Haiku, the only difference is that its running Swift on my iPhone instead of my iPad. MyChart and Hyperspace are different. There’s a superficial difference, which is that they are clients that don’t run on iOS/iPadOS devices. But there’s a deeper difference, which is how the user’s device gets access to the client code.
That’s the tricky part. Its also related to Jacob’s tech issue. Getting access to the Haiku or Canto client is fairly straightforward. They are apps that you can download from the Apple (or Google) App(Play)Store. You download the code, its on your iDevice, if Epic wants to push an update they can upload a new version to the AppStore, and Apple will take care of updating the code on your iDevice. MyChart and Hyperspace are different, very different. One can think of a couple reasons why they might be different. But in my mind primary driver of the differences is time. All of these clients were introduced slowly over time and each one follows the primary client deployment paradigm of the time they were developed in. Walking backward through time in a very simplistic manner: the AppStore was a big deal when it came out in 2008, it upset the web-based paradigm of the early 2000s. The 2000’s web-based paradigm itself had taken over from the locally installed application paradigm of the ‘90s. MyChart follows the web paradigm and Hyperspace follows the locally installed paradigm.
The web paradigm is sort of cool because the idea is that client code is sent to the users device just-in-time. It is also how all websites work. When you you tell your browser to go to your favorite website, the browser gets a bunch of code from that website. That code package is made up of HTML, CSS, and Javascript and tells your browser what to show and how to interact with the back-end. Since the client code is requested when you visit the site, front-end developers do not need to worry about pushing updates to multiple devices. They just need to update the server that serves the front-end code. From that point on all users that visit the site will get access to the latest and greatest code. Pretty slick, because you don’t need an Apple-like middle man to keep everyone’s client code up to date. MyChart for the most part works like this. Its not quite as straightforward because MyChart is tied to each health-system that uses it, so the updates from Epic will need to go through them in order to be seen by patients.
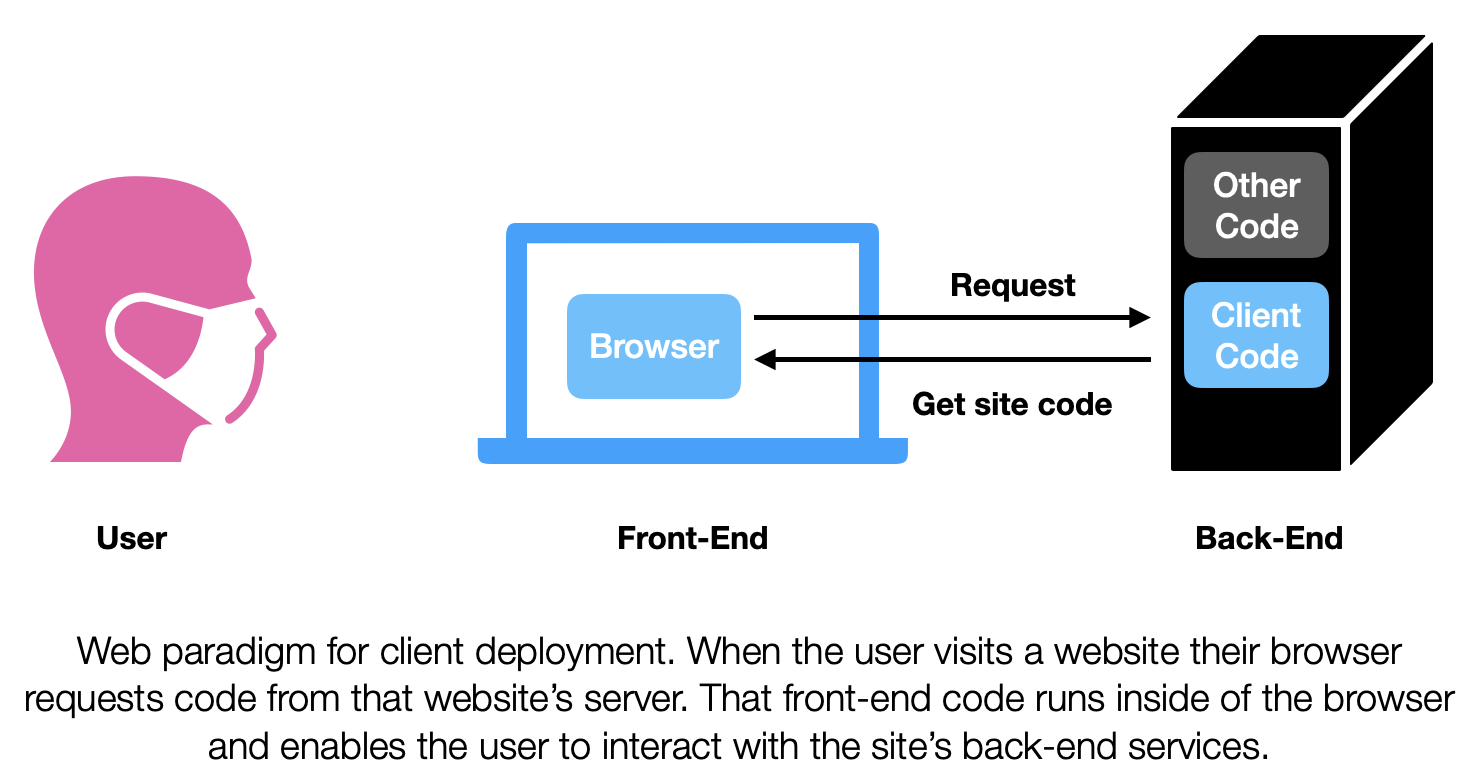
The web paradigm is sort of cool because the idea is that client code is sent to the users device just-in-time. It is also how all websites work. When you you tell your browser to go to your favorite website, the browser gets a bunch of code from that website. That code package is made up of HTML, CSS, and Javascript and tells your browser what to show and how to interact with the back-end. Since the client code is requested when you visit the site, front-end developers do not need to worry about pushing updates to multiple devices. They just need to update the server that serves the front-end code. From that point on all users that visit the site will get access to the latest and greatest code. Pretty slick, because you don’t need an Apple-like middle man to keep everyone’s client code up to date. MyChart for the most part works like this. Its not quite as straightforward because MyChart is tied to each health-system that uses it, so the updates from Epic will need to go through them in order to be seen by patients.
Finally we get to Hyperspace. Hyperspace, by nature of being Epic’s most capable client is also its most complicated client. The internal complexity of Hyperspace was what I was thinking about when I was troubleshooting with Jacob. Despite this internal complexity Hyperspace has the potential to be the simplest client to deploy. As mentioned above, it uses the locally installed paradigm. Every child of the 90s should be familiar with this paradigm; you find an a program you want to use from the internet (or get a cd), download the executable, run through then installation process (🧙🏽♂️). Then you use the downloaded program to your heart’s content. That’s the paradigm that Hyperspace was designed for. In the early 2000s, at the time of my high school sports physical, that was the paradigm that was used. When my doc launched Hyperspace, he was running code that was installed on computer sitting in the room with us. When a new clinic was to be set up all of the computers going there needed to have Hyperspace installed on them. When Hyperspace was updated all of the computers in all of the clinics and wards needed to have their software updated. Additionally, installing and running hyperspace locally on all these computers meant that all the computers needed to meet all the requirements needed in terms of RAM and compute power.
As you can see, installing and using Hyperspace entirely locally is problematic. The deployment management perspective alone is headache inducing. And what if people want to access the EHR from home? Users would need to install Hyperspace on their own machines? And need to keep them up to date? Forget about it! The solution to these headaches is brilliant in a way. Hyperspace needs to run on a windows computer, but that computer doesn’t need to physically exist in the clinic as long as the people in the clinic can virtually access that computer. Enter virtualization.
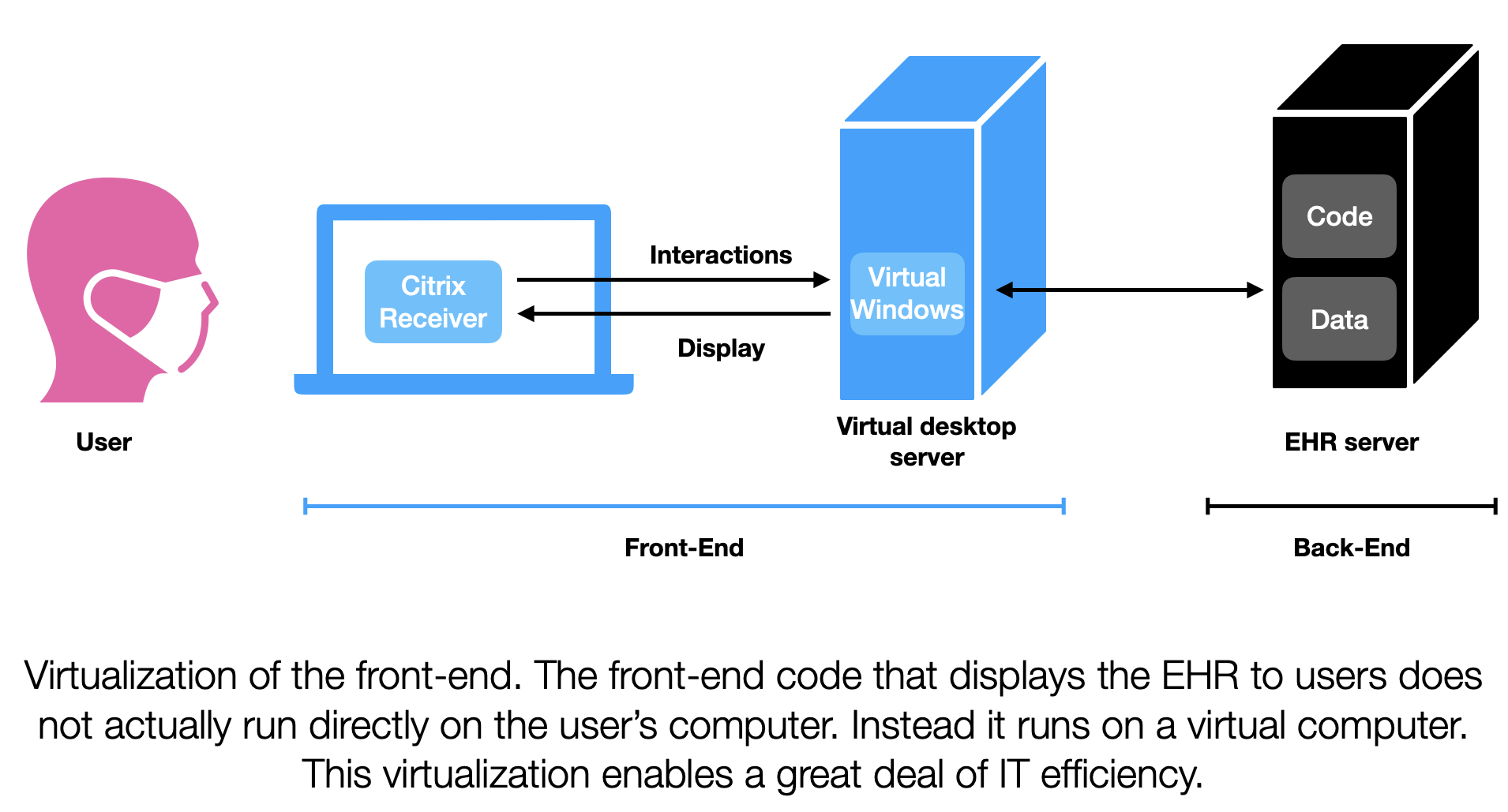
Virtualization, specifically desktop virtualization is best described by Wikipedia: “desktop virtualization is a software technology that separates the desktop environment and associated application software from the physical client device that is used to access it.” [4] What it enables is moving all of those individual computers (and the Hyperspace client) to virtual Windows servers. Then all the computers in the clinic need to do is to connect to those servers. Those virtual Windows servers will then present the whole desktop experience to the users. Maintaining virtual Windows computers is a lot easier than maintaining physical Windows computers. Updating software on those virtual computers is a lot easier too. In the late 2000s Citrix released software that enabled businesses to have virtual desktops and for other computers to connect to those virtual desktops (Citrix Receivers, AKA Citrix Workspace App). [5] If packaged properly, you won’t even notice that you’ve launched into another computer, you will just see the application you are interested in using. This is what currently happens with Hyperspace.
So Hyperspace went from being installed locally on the computers in clinic to being installed locally on a virtual Windows computer that you access from clinic (or home). The way you access the Hyperspace client is through another client, the Citrix Receiver. This Russian nesting doll setup has added some complexity but greatly also greatly simplified deployment headaches. Using virtualization is pretty cool because it allows locally installed clients to be deployed in a manner analogous to web-based deployment. You end up trading off one type of complexity (managing lots of local installations) with another (maintaining virtualization), but on the whole it’s a good trade for IT departments.
What of Jacob’s issue? Well it turns out it was a Citrix Receiver issue. As a client Citrix Receiver takes your mouse and keyboard inputs sends them to the server running Windows and Hyperspace virtually. This virtual computer returns what should be displayed and Citrix Receiver displays it. Some time before Jacob called me, Citrix Receiver had updated and asked if Jacob would like to update his resolution settings, he had inadvertently said yes. This in turn made the fonts on Hyperspace appear really tiny. Reverting that setting helped return the Hyperspace display to normal.
When Jacob told me about the fix and how it involved changing a Citrix Receiver setting I kicked myself. Its the one part of the system I would never think to check. It was a good reminder that there’s a lot of complexity built into every part of the system that serves our patient records. While I spend most of my time thinking about other parts of the EHR this bug was a good reminder to not forget about the humble client.
Erkin
[Go ÖN Home](../../index.md)
## Acknowledgements
I’d like to thank [John Cheadle](https://www.linkedin.com/in/john-cheadle-59774339) and [River Karl](https://www.linkedin.com/in/river-karl-7b5a4349) for reviewing this work prior its posting.
## Bibliography
1. Frontend and backend - Wikipedia. 2022; Available from: https://en.wikipedia.org/wiki/Frontend_and_backend.
2. Client–server model - Wikipedia. 2022; Available from: https://en.wikipedia.org/wiki/Client%E2%80%93server_model.
3. JEFF GLAZE jglaze@madison.com, -.-. Epic Systems draws on literature greats for its next expansion. 2022.
4. Desktop virtualization - Wikipedia. 2022; Available from: https://en.wikipedia.org/wiki/Desktop_virtualization.
5. Citrix Workspace App - Wikipedia. 2022; Available from: https://en.wikipedia.org/wiki/Citrix_Workspace_App.
------------------------
File: 2022-02-15-needle-gauges.md
Creation Date: "Sat, 27 Jul 2024 12:20:28 +0000"
---
title: "Bigger Needle Smaller Number"
categories:
- Blog
tags:
- engineering
- industrial engineering
- medicine
- hypodermic needles
- gauges
- measurement system
- industrialization
- standards
header:
teaser: "/assets/images/insta/IMG_4191.JPG"
overlay_image: "/assets/images/insta/IMG_4191.JPG"
---
This is going to be a short post because the last one about EHR front-ends was about 3 times longer than I had originally planned for it to be.
A while ago I came across this wonderful tweetorial on the history of needle gauges. It is a summary of an article entitled “The story of the gauge” by Pöll. [1] Pöll traces the lineage of the Birmingham Wire Gauge (BWG) system (the measurement system we use to describe the diameter of the hypodermic needles). Its an interesting story that lays out how we ended up using a seemingly counterintuitive system developed in the 19th century to communicate the size of needles we want to use.
As a med student we are taught to ask for “two-large bore” IVs when a patient is at risk of needing a large amount of blood or fluid transfused. My notes say this is 16 gauge or larger (I’ve seen 18 or larger as well). The “larger” part can be confusing when it comes to needle gauges. [2] This is because larger needle diameters actually have smaller gauge numbers.
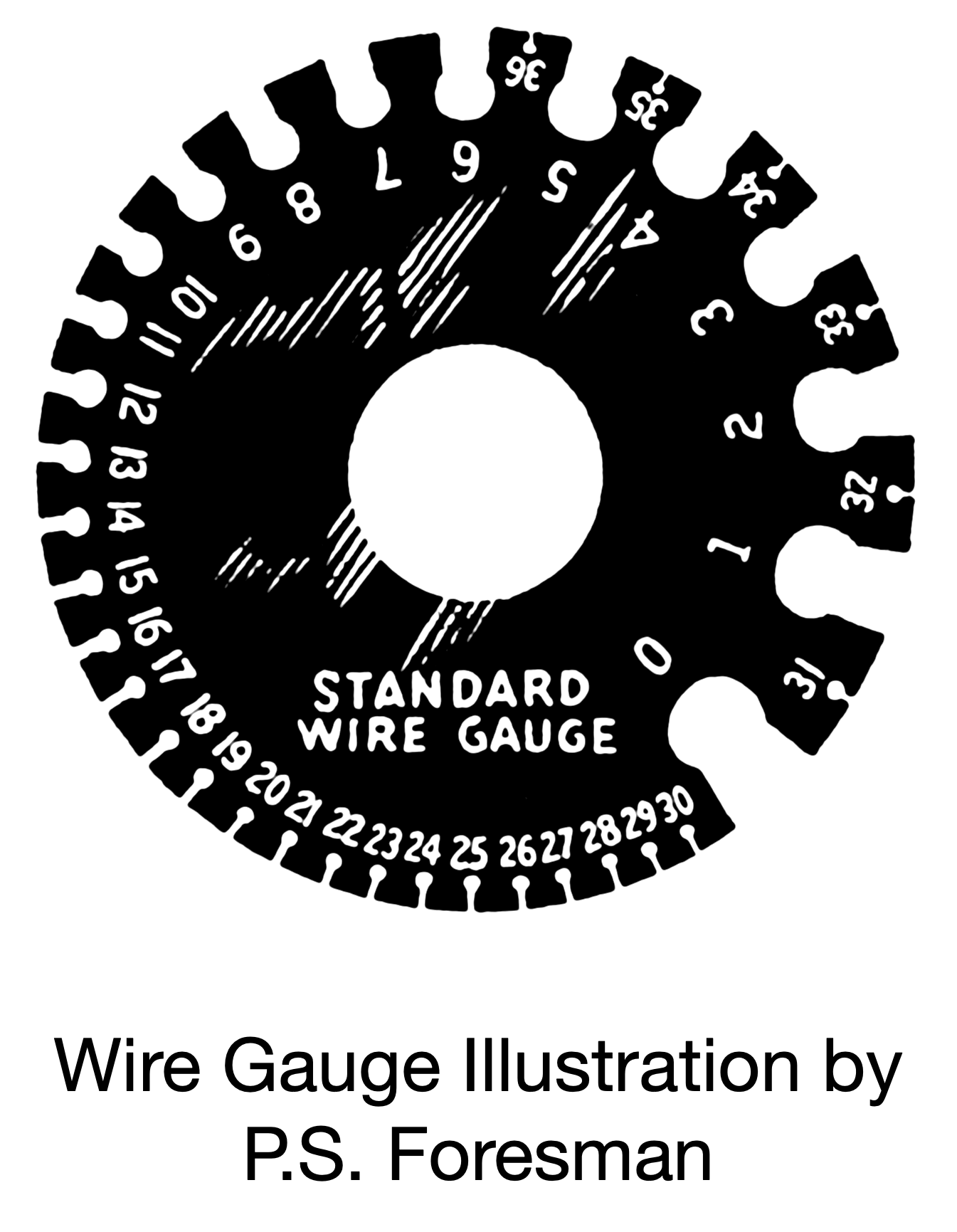
The reason for this comes down to development of the BWG. It was developed to measure the thinness of drawn wire. Wire is drawn (or made thinner) by pulling metal through dies (holes in metal templates). You make the wire thinning by repeatedly drawing it through smaller holes. The numbering of these holes is the gauge. Thus the larger the gauge the thinner the wire (or needle).
Reading through the history of how the BWG came to be the standard for wire (and needle) gauging is a good reminder that standards and nomenclature don’t emerge linearly in relation to the technology being used. I think this is especially true in healthcare where technology often gets ported after being developed elsewhere.
Erkin
[Go ÖN Home](../../index.md)
P.S. There are some really cool physical properties that interplay with gauge size. One has to do with intermolecular forces (van Der Waals forces), which lead to a neat relationship between the gauge sizes, each gauge is about 11% thinner than preceding gauge. [1] The second has to do with the flow rate through a needle which is related to the quadratic power of the radius of a needle. [2]
## Bibliography
1. Pöll, J.S., The story of the gauge. Anaesthesia, 1999. 54(6): p. 575-581.
2. Verhoeff, K., et al., Ensuring adequate vascular access in patients with major trauma: a quality improvement initiative. BMJ Open Quality, 2018. 7(1): p. e000090.
------------------------
File: 2022-02-17-Early-identification-of-patients-admitted-to-hospital-for-covid-19-at-risk-of-clinical-deterioration.md
Creation Date: "Sat, 27 Jul 2024 12:20:28 +0000"
---
title: "Early identification of patients admitted to hospital for covid-19 at risk of clinical deterioration: model development and multisite external validation study"
categories:
- Blog
- Research
tags:
- Blog
- Research
- covid
- deterioration index
- early warning system
- medicine
- healthcare
- artificial intelligence
- machine learning
header:
teaser: "/assets/images/insta/IMG_2184.JPG"
overlay_image: "/assets/images/insta/IMG_2184.JPG"
---
British Medical Journal. Can be found [here](https://doi.org/10.1136/bmj-2021-068576).
[Download paper.](https://eotles.com/assets/papers/early_identification_of_covid_inpatients_at_risk_of_clinical_deterioration.pdf)
## Abstract
### Objective
To create and validate a simple and transferable machine learning model from electronic health record data to accurately predict clinical deterioration in patients with covid-19 across institutions, through use of a novel paradigm for model development and code sharing.
### Design
Retrospective cohort study.
### Setting
One US hospital during 2015-21 was used for model training and internal validation. External validation was conducted on patients admitted to hospital with covid-19 at 12 other US medical centers during 2020-21.
### Participants
33,119 adults (≥18 years) admitted to hospital with respiratory distress or covid-19.
### Main outcome measures
An ensemble of linear models was trained on the development cohort to predict a composite outcome of clinical deterioration within the first five days of hospital admission, defined as in-hospital mortality or any of three treatments indicating severe illness: mechanical ventilation, heated high flow nasal cannula, or intravenous vasopressors. The model was based on nine clinical and personal characteristic variables selected from 2686 variables available in the electronic health record. Internal and external validation performance was measured using the area under the receiver operating characteristic curve (AUROC) and the expected calibration error—the difference between predicted risk and actual risk. Potential bed day savings were estimated by calculating how many bed days hospitals could save per patient if low risk patients identified by the model were discharged early.
### Results
9291 covid-19 related hospital admissions at 13 medical centers were used for model validation, of which 1510 (16.3%) were related to the primary outcome. When the model was applied to the internal validation cohort, it achieved an AUROC of 0.80 (95% confidence interval 0.77 to 0.84) and an expected calibration error of 0.01 (95% confidence interval 0.00 to 0.02). Performance was consistent when validated in the 12 external medical centers (AUROC range 0.77-0.84), across subgroups of sex, age, race, and ethnicity (AUROC range 0.78-0.84), and across quarters (AUROC range 0.73-0.83). Using the model to triage low risk patients could potentially save up to 7.8 bed days per patient resulting from early discharge.
### Conclusion
A model to predict clinical deterioration was developed rapidly in response to the covid-19 pandemic at a single hospital, was applied externally without the sharing of data, and performed well across multiple medical centers, patient subgroups, and time periods, showing its potential as a tool for use in optimizing healthcare resources.
------------------------
File: 2022-02-21-call-tech-support.md
Creation Date: "Sat, 27 Jul 2024 12:20:28 +0000"
---
title: "Why Doctors Should Contact Tech Support"
categories:
- Blog
tags:
- tech support
- health IT
- healthcare
- medicine
- enterprise software
header:
teaser: "/assets/images/insta/IMG_2025.JPG"
overlay_image: "/assets/images/insta/IMG_2025.JPG"
---
> If you see something, say something.
This post is a manifesto on reporting bad health information technology (HIT) problems.
If you’re having HIT problems complain about ‘em! I know everyone in medicine is conditioned not to complain and to deal with the crappiness of the “system”. But HIT is an area of healthcare where you can make a difference just by complaining.
While a lot of the problems in HIT run pretty deep (*cough* usability *cough*) there are many things that can be fixed if attention is brought to them. These are things like:
changing the order of columns on the team patient sign-off/hand-off report,
stopping a best practice alert that no longer matches your clinical practice, or
improving the loading time of a patient’s chart.
None of these are big changes that involve redesigning user-interfaces or re-factoring server-side code. They are simple changes that will make the task of using HIT less arduous. If you put in a help-desk ticket with your hospital’s HIT team its very likely that they can fix the issue quickly and slightly improve your experience.
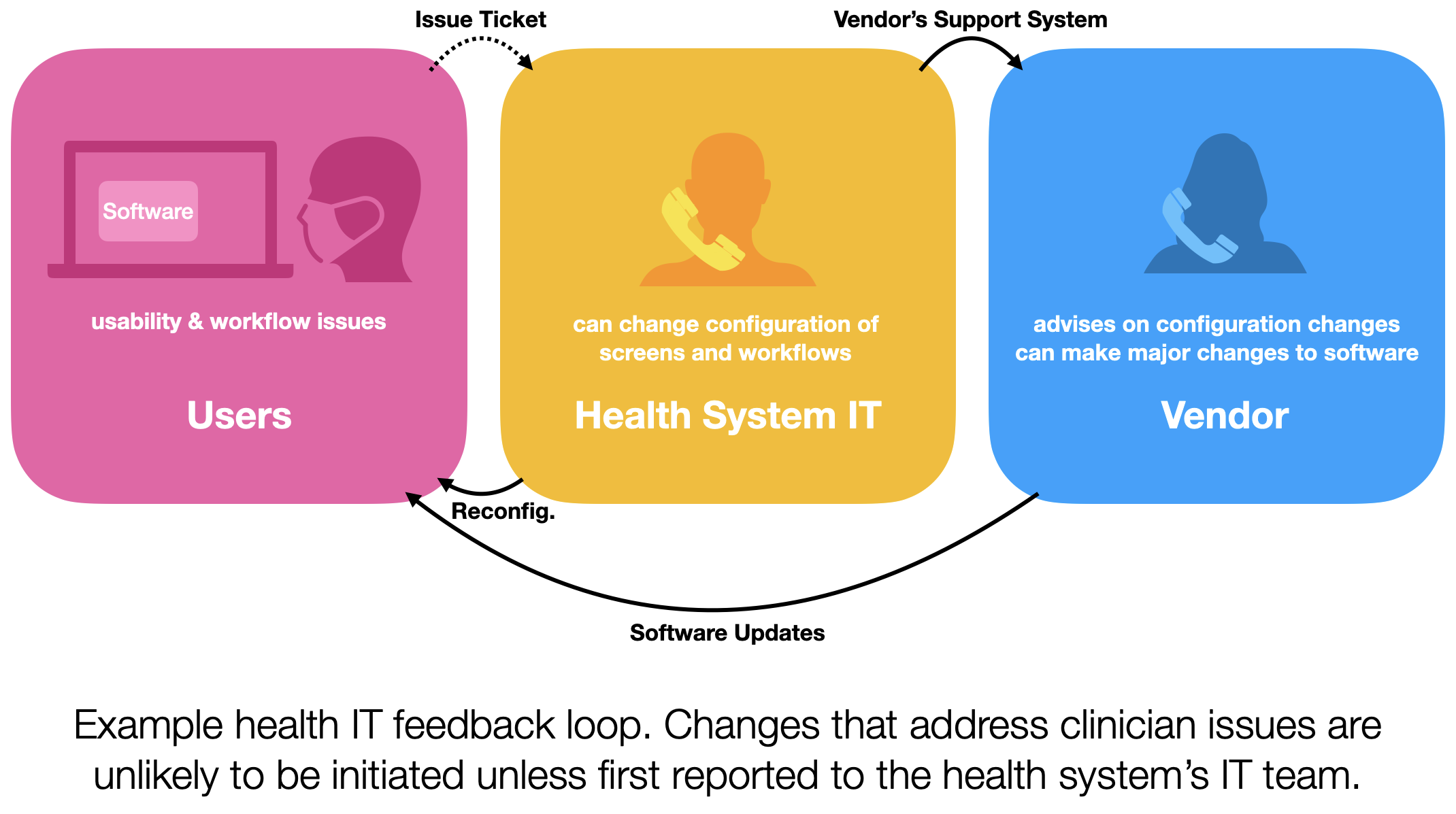
You might say “well I don’t do that with any of the other software I use” and that’s true. I don’t think I’ve ever reached out to tech support for Microsoft Word, iOS, or Instagram. There’s a couple reasons for this, but the one most relevant to our discussion is feedback. The developers of most consumer software may actually USE their software on a daily basis. So there’s a very tight feedback loop. With healthcare IT this feedback loop is long and leaky.
Let’s take the electronic health records (EHRs). Most EHR systems are sold to health systems as enterprise software. That is software that one company sells (or licenses) to another company (the health system). The health system then has their clinicians use the EHR. This setup means that there are several tiers of support for the software. Additionally the software company specializes in making software, not using it, so their developers may not have a good sense of how the software works “in the wild”. Contrast this with a developer at Slack, who may use Slack to interact with their coworkers. User feedback doesn’t naturally occur in the EHR development space. So what do we do?
We use the system! There’s a feedback loop built in for us, but its not widely known. That feedback loop is initiated by reporting issues. When a doctor or nurse reports an issue to their health system’s HIT team that should kick-off the feedback process. Your issue ticket will be triaged and then sent to the people who can fix it, either the HIT team or the software vendor. Neither of those teams are going to do anything for you if you don’t tell them what’s wrong.
So report your issues. Your HIT team might fix them. Your software vendor might make an improvement in the future. Your work tech life might get an iota better and your colleagues might thank you. Sure there’s a lot of “mights”. But these things won’t happen if you don’t say something first.
Erkin
[Go ÖN Home](../../index.md)
P.S. while writing this I found myself mulling over the bad tech support experiences I’ve had in the past. As someone who was essentially in tech support I’ve developed some techniques that I can share in another post if people are interest. Additionally, tech support for HIT should not be a blackhole, if it is that’s a red flag and should be rectified. Stifling this feedback loop is a surefire way to miss critical safety issues.
------------------------
File: 2022-03-01-intro-to-ml-part-i.md
Creation Date: "Sat, 27 Jul 2024 12:20:28 +0000"
---
title: "Intro to Machine Learning Models for Physicians: Part I"
categories:
- Blog
tags:
- machine learning
- artificial intelligence
- operations research
- statistics
- healthcare
- medicine
header:
teaser: "/assets/images/insta/IMG_1613.JPG"
overlay_image: "/assets/images/insta/IMG_1613.JPG"
---
This a foundational post that has two aims. The first is to demystify machine learning which I believe is key to enabling physicians and other clinicians to become empowered users of the machine learning tools they use. There’s a bit of ground I want to cover, so this post will be broken into several parts. This part situates and introduces machine learning then discusses the important components of machine learning models.
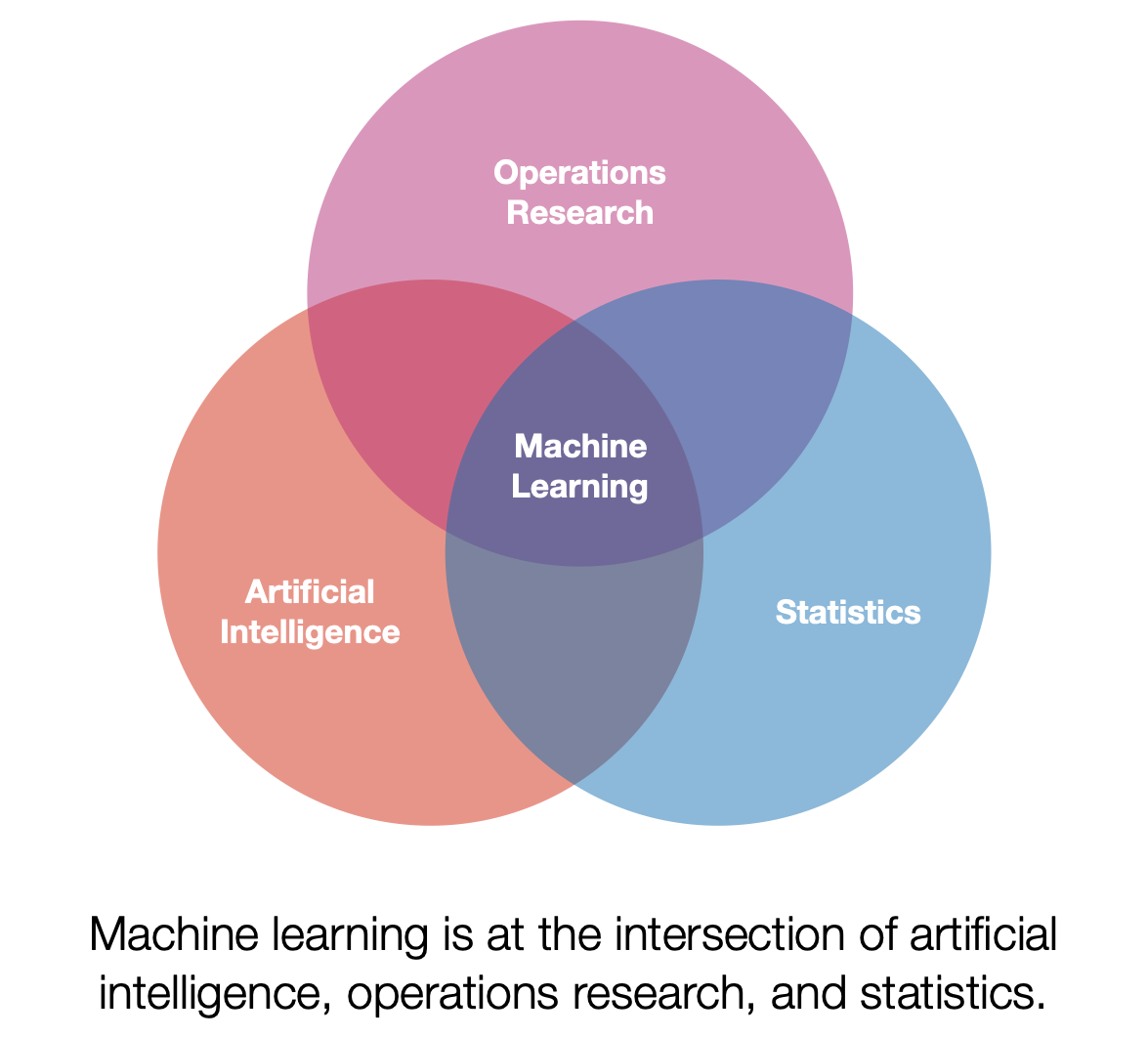
## An Introduction
First a note on terminology. Machine learning (ML) can mean a lot of different things depending on who you ask. I personally view ML as a subset of artificial intelligence that has a strong focus on using data to build models. Additionally, ML has significant overlaps with operations research and statistics. One of my favorite definitions of ML models is presented by Tom Mitchell. [1] Paraphrased below:
> A model is said to learn from experience if its performance at a task improves with experience.
Quick note, the term _model_ will be more fully explained below.
This set up lends itself well to analogy. One potential analogy is that of a small child learning how to stack blocks. The child may start from a point where it is unable to stack blocks, it will repeatedly attempt stacking, and eventually will master how to stack blocks in various situations. In this analogy stacking blocks is the task, the repeated attempts at stacking is the experience, and the performance is some criteria the child uses to assess how well they are stacking (e.g., height or stability).
We will now discuss this general definition for the specific use case of ML for healthcare. To contextualize this discussion we will focus on the ML model types that are most widely used in healthcare, _supervised offline learning_.[^1] Let’s break it down bit by bit. First, _supervised learning_ constrains the learning process by introducing supervisory information, this information can be thought of as a teacher that tells the model if they got the task correct. This is very useful when trying to evaluate the performance of the model. In addition to being supervised the models used for healthcare are often developed in an _offline_ setting. Offline describes the manner in which the model gains experience. Instead of learning from direct interaction with their environment they gain their experience by using information that has already been collected.
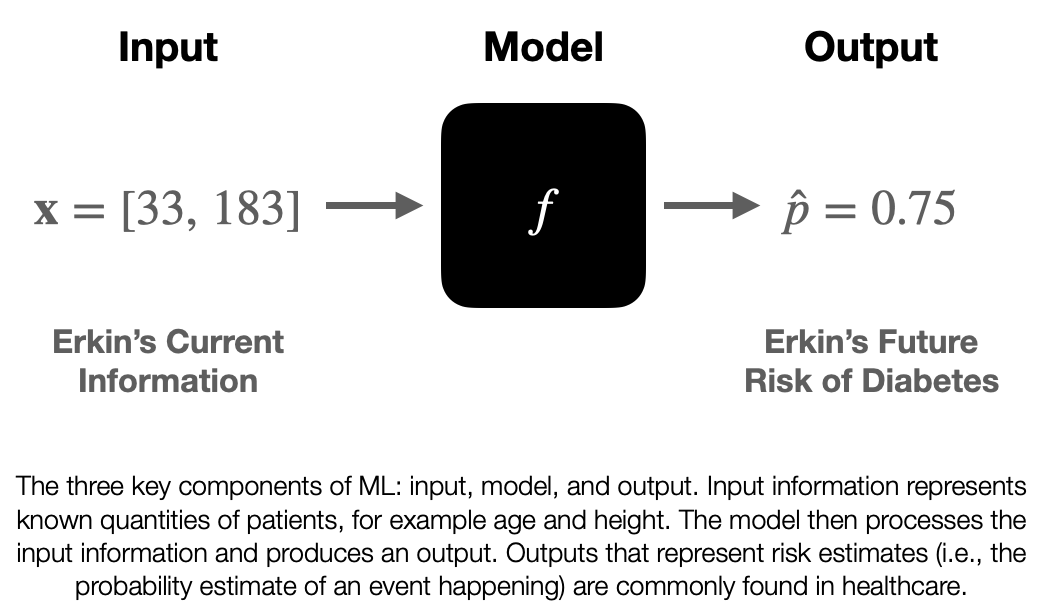
## What is an ML model?
We’ve been talking about the concept of the model pretty abstractly, so let’s nail it down now.
A model is a mathematical function, f, that operates on information, taking in input information and returning output information. This function f is the thing that “learns from experience”, however in our case the function has stopped learning by the time it is ready to be used. So when it is implemented in an EHR system f is usually fixed. We will discuss how f is created in the next blog post, but for now let’s treat it like a black box and discuss the information it interacts with.
The input information is known as **x**. Unlike the **x** you were introduced to in algebra class it actually represents information that we know. This information can take different forms depending on what information represents, but it is common to see **x** represent a list (or vector) of numbers. For example, if we wanted to give a model my age and height as input information you could set **x**=[33, 183], where 33 is my age in years and 183 is my height in centimeters.
The output of a model may vary based on use-case and may be a little opaque. I’ll present my notation (which may differ from what you see elsewhere), I believe this is notation is the easiest to understand. In healthcare we are often interested in risk stratification models that output risk estimates, denoted as (pronounced: p-hat). Risk estimates are estimates of the probability that an event will happen to a given patient. Let’s say we have a model that can assess a patient’s risk of developing diabetes in the next decade. If given information about me the model returns a we could then say that the model estimates my risk of developing diabetes in the next decade as 75%. Ultimately should be a value between 0 and 1. By returning a numerical value along a continuous scale this is a type of regression (just like linear regression from high school statistics).
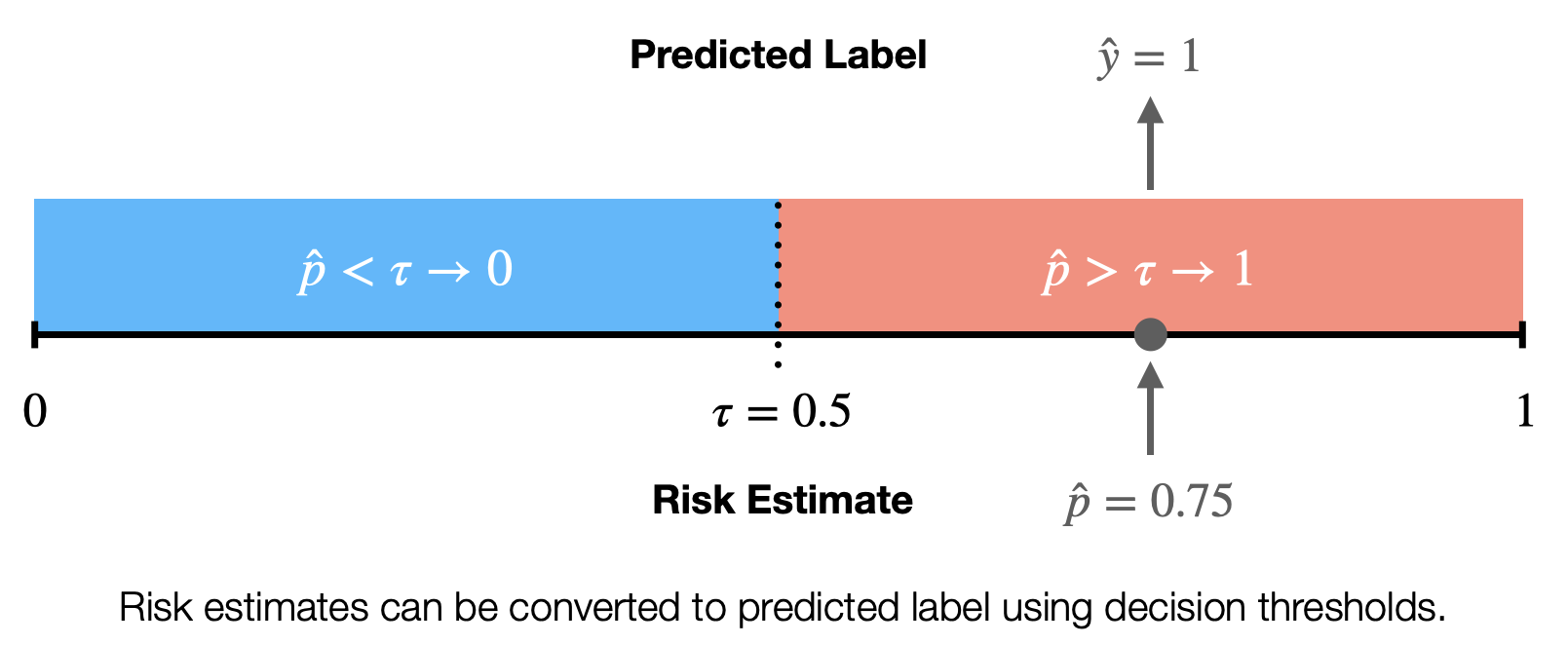
Sometimes we want to use models to separate out different populations of patients, for example to tell us if a patient belongs to the high-risk or low-risk group. When we use the model to return this information we call that output the predicted label. We denote predicted labels as (y-hat). We will loop back on a discussion of labels, but for now you can think of them as a model assigned group. This is a type of classification, specifically binary classification, which splits patients into two groups. We can convert a regression model into a classification model by employing a decision threshold. The decision threshold, (tau), is a number between 0 and 1 that can be used to split the risk estimates into two discrete categories. For example we set could set for the diabetes model mentioned above and say that all risk estimates greater than correspond to a high-risk of developing diabetes (). So a decision threshold can be used to transform the risk estimates into predicted labels.
Most of the ML systems used in clinical practice use a model, inputs, and outputs in a manner similar to what we’ve discussed. For example the Epic Sepsis Model can be thought of in these terms. Every 15 minutes the model receives input information, summarizing key fields from the EHR (such as vital signs, lab values, and medication orders). The model then does some basic math (you could do the math on a calculator if you were very patient) and returns a value between 0 and 100. These output values are then compared against a decision threshold and if the patient’s output is greater than the decision threshold (e.g., Michigan uses 6) then something happens (like paging a nurse about the patient being high risk). [2]
Understanding the components of ML models is important because it helps to demystify the functioning of the models and the overall process. There may be black boxes involved, but the input and outputs flanking the model should be familiar to physicians. In the coming post we will discuss how ML models are built. This will then eventually be followed by a discussion of how ML models are deployed.
Erkin
[Go ÖN Home](../../index.md)
[^1]: Note ML is not a monolith and there are many different techniques that fall under the general umbrella of ML and I may cover some of the different types of ML in another post (e.g. unsupervised and reinforcement learning).
## Bibliography
1. Mitchell, T.M., Machine Learning. McGraw-Hill series in computer science. 1997, New York: McGraw-Hill. xvii, 414 p.
2. Wong, A., et al., External Validation of a Widely Implemented Proprietary Sepsis Prediction Model in Hospitalized Patients. JAMA Internal Medicine, 2021.
### Footnotes
------------------------
File: 2022-03-07-doctors-notes-software-prototype.md
Creation Date: "Sat, 27 Jul 2024 12:20:28 +0000"
---
title: "Doctor’s Notes Software Prototype"
categories:
- Blog
- Project
tags:
- health IT
- doctor’s notes
- electronic health records
- software design
- UI/UX
- human factors engineering
excerpt: "A project that was focused on examining and improving the way doctor’s notes are written."
header:
teaser: "/assets/images/insta/IMG_1087.JPG"
overlay_image: "/assets/images/insta/IMG_1087.JPG"
---
We will return to the “Intro to ML for Physicians” series next week. In the intervening time here’s a short post about a prototype health IT app I made a two years ago. I made this app as part of a team project that was focused on examining and improving the way doctor’s notes are written.
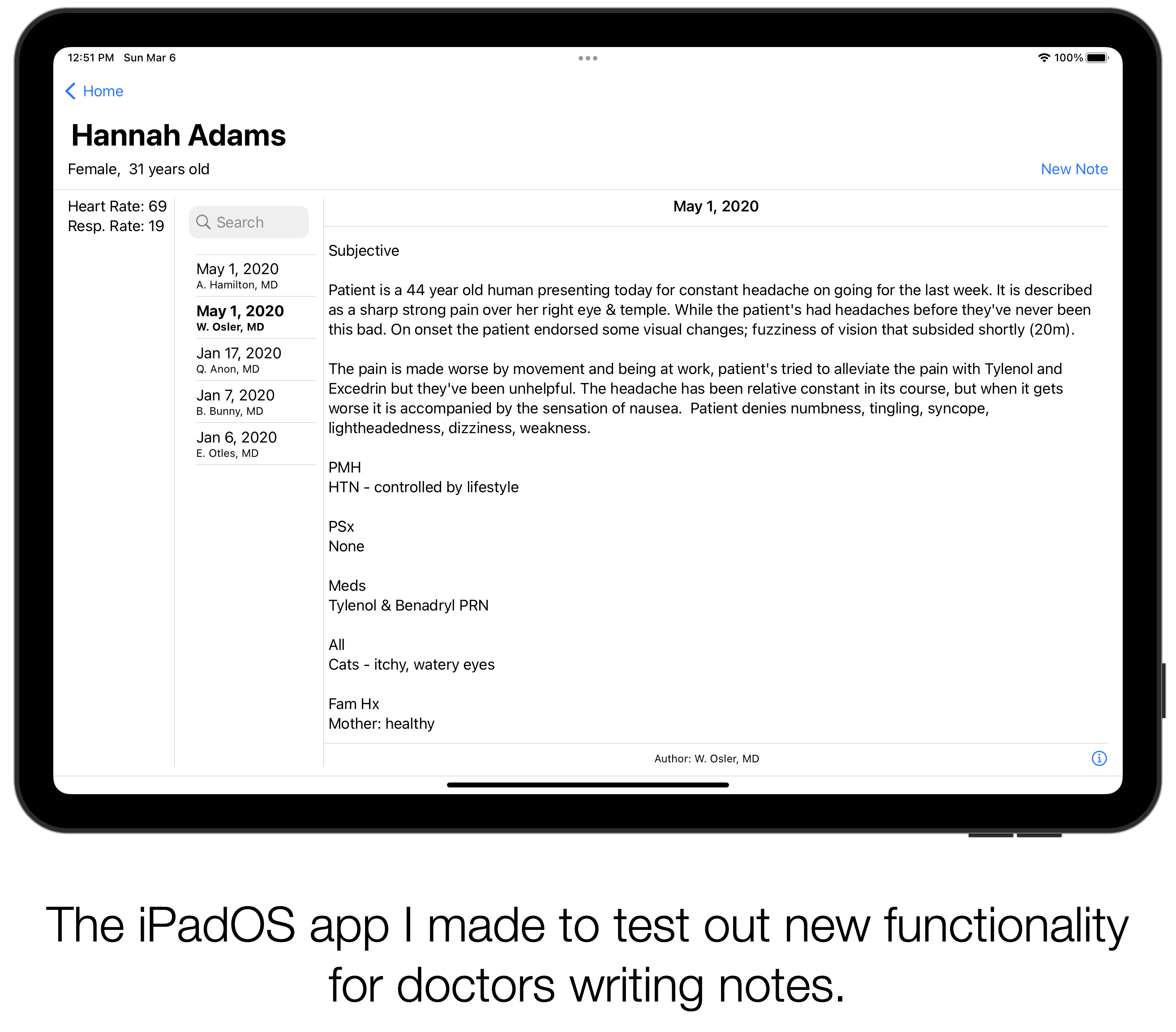
Nominally this was a graduate project (holler at my HCI team[^1]) and the project specification called for making a low-functionality prototype using invision. [1], We did this and found it unsatisfying. The reason for this was that we wanted to incorporate a voice transcription interface into the note writing process. Although we could replicate some of the other functionality there was no way to build voice transcription and other key functionality in the prototyping software.
So I took the logical nextstep[^2] and built out a minimal viable prototype using Apple’s development tools. This allowed me to incorporate on-device transcription. [2, 3] On-device transcription is a really cool technology for healthcare IT! Because you don’t have information flowing off the device back to Apple’s (or someone else’s) servers, it could enable HIPAA compliant voice interfaces in the future. Making a prototype app also enabled me to build several other features, such as saving and retrieving notes. These features are necessary when testing out a more complicated record keeping system, like this.
If you are interested in learning more about this prototype check out this video:
If you would like to take a look at my hacky Swift code check out the [Github project](https://github.com/eotles/HCI).
One thing that I didn’t have time to code up was the sharing of notes between physicians. This is a pain point in systems that are actually in use. The team had some cool ideas about collaborative editing and version control. I think these would be super useful from both a clinical perspective (making the sharing, editing, and co-signing easier) and also from a technical perspective. However that would involve a significant amount of back-end development (see: [Complicated Way You See Patient Data: EHR Front-Ends](https://eotles.github.io/blog/posts/20220206_ehr_front_ends/)) so it remains an item todo.
One of my mantras is that there’s a lot of work to be done in healthcare IT. Developing prototypes and testing them out can help us advance the state of the field. Rapidly prototyping these systems is hard to do, but it could pay dividends in terms of physician happiness and productivity.
Erkin
## P.S.
Although I’ve made a couple other apps using Xcode and Swift this was my first time using SwiftUI, which was a pretty slick experience.[4] I really enjoyed programmatically creating the interface and not having to toggle back and forth between my view controller code and the Interface Builder.
## Acknowledgements
I’d like to thank the team: [Sarah Jabbour](https://sjabbour.github.io), [Meera Krishnamoorthy](http://meera.krishnamoorthy.com), [Barbara Korycki](https://www.linkedin.com/in/barbara-korycki-19568810a/), and [Harry Rubin-Falcone](https://www.linkedin.com/in/harry-rubin-falcone-a6543960/). Making wireframes with you guys was an absolute joy.
## Bibliography
1. Prototype | InVision. 2022; Available from: https://www.invisionapp.com/defined/prototype.
2. Bolella, D. SpeechTranslatorSwiftUI Github Project. Available from: https://github.com/dbolella/SpeechTranslatorSwiftUI.
3. Recognizing Speech in Live Audio | Apple Developer Documentation. 2022; Available from: https://developer.apple.com/documentation/speech/recognizing_speech_in_live_audio.
4. SwiftUI Tutorials | Apple Developer Documentation. 2022; Available from: https://developer.apple.com/tutorials/swiftui.
### Footnotes
[^1]: Sarah Jabbour, Meera Krishnamoorthy, Barbara Korycki, and Harry Rubin-Falcone
[^2]: kudos if you got the joke
------------------------
File: 2022-04-02-AR-example.md
Creation Date: "Sat, 27 Jul 2024 12:20:28 +0000"
---
title: "Augmented Reality Demo"
categories:
- Blog
tags:
- AR/VR/XR
---
This is an augmented reality (AR) demo using Apple's Augmented Reality tools. The 3D asset is a USDZ file created by [Apple](https://developer.apple.com/augmented-reality/quick-look/) (they own all rights to it). It is hosted as file uploaded to this GitHub repository.
[Click this link to check it out.](https://github.com/eotles/blog/blob/gh-pages/posts/20220402_AR_example/toy_biplane.usdz?raw=true) It will download the file to your device. If it is an iOS device it should automatically open up the AR Quick Look functionality.
Erkin
[Go ÖN Home](../../index.md)
------------------------
File: 2022-08-29-Dynamic-prediction-of-work-status-for-workers-with-occupational-injuries.md
Creation Date: "Sat, 27 Jul 2024 12:20:28 +0000"
---
title: "Dynamic prediction of work status for workers with occupational injuries: assessing the value of longitudinal observations"
categories:
- Blog
- Research
tags:
- Blog
- Research
- occupational health
- return to work
- medicine
- healthcare
- artificial intelligence
- machine learning
header:
teaser: "/assets/images/insta/IMG_1609.JPG"
overlay_image: "/assets/images/insta/IMG_1609.JPG"
---
Journal of the American Medical Informatics Association manuscript, can be found [here](https://doi.org/10.1093/jamia/ocac130).
[Download abstract.](https://eotles.com/assets/papers/dynamic_prediction_of_work_status_for_workers_with_occupational_injuries.pdf)
## Abstract
### Objective
Occupational injuries (OIs) cause an immense burden on the US population. Prediction models help focus resources on those at greatest risk of a delayed return to work (RTW). RTW depends on factors that develop over time; however, existing methods only utilize information collected at the time of injury. We investigate the performance benefits of dynamically estimating RTW, using longitudinal observations of diagnoses and treatments collected beyond the time of initial injury.
### Materials and Methods
We characterize the difference in predictive performance between an approach that uses information collected at the time of initial injury (baseline model) and a proposed approach that uses longitudinal information collected over the course of the patient’s recovery period (proposed model). To control the comparison, both models use the same deep learning architecture and differ only in the information used. We utilize a large longitudinal observation dataset of OI claims and compare the performance of the two approaches in terms of daily prediction of future work state (working vs not working). The performance of these two approaches was assessed in terms of the area under the receiver operator characteristic curve (AUROC) and expected calibration error (ECE).
### Results
After subsampling and applying inclusion criteria, our final dataset covered 294 103 OIs, which were split evenly between train, development, and test datasets (1/3, 1/3, 1/3). In terms of discriminative performance on the test dataset, the proposed model had an AUROC of 0.728 (90% confidence interval: 0.723, 0.734) versus the baseline’s 0.591 (0.585, 0.598). The proposed model had an ECE of 0.004 (0.003, 0.005) versus the baseline’s 0.016 (0.009, 0.018).
### Conclusion
The longitudinal approach outperforms current practice and shows potential for leveraging observational data to dynamically update predictions of RTW in the setting of OI. This approach may enable physicians and workers’ compensation programs to manage large populations of injured workers more effectively.
------------------------
File: 2022-08-30-IOE-RTW-JAMIA-Press.md
Creation Date: "Sat, 27 Jul 2024 12:20:28 +0000"
---
title: "Helping people get back to work using deep learning in the occupational health system"
categories:
- Blog
- Press
tags:
- Blog
- Press
- occupational health
- return to work
- medicine
- healthcare
- artificial intelligence
- machine learning
header:
teaser: "/assets/images/insta/IMG_1408.JPG"
overlay_image: "/assets/images/insta/IMG_1408.JPG"
---
Discussed our recent [JAMIA paper on predicting return to work](/blog/research/Dynamic-prediction-of-work-status-for-workers-with-occupational-injuries/) with Jessalyn Tamez. Check out the news brief [here](https://ioe.engin.umich.edu/2022/08/30/helping-people-get-back-to-work-using-deep-learning-in-the-occupational-health-system/).
------------------------
File: 2022-09-19-Prospective-evaluation-of-data-driven-models-to-predict-daily-risk-of-clostridioides-difficile-infection-at-2-large-academic-health-centers.md
Creation Date: "Sat, 27 Jul 2024 12:20:28 +0000"
---
title: "Prospective evaluation of data-driven models to predict daily risk of Clostridioides difficile infection at 2 large academic health centers"
categories:
- Blog
- Research
tags:
- Blog
- Research
- Clostridioides difficile
- infectious disease
- early warning system
- medicine
- healthcare
- artificial intelligence
- machine learning
header:
teaser: "/assets/images/insta/IMG_1144.JPG"
overlay_image: "/assets/images/insta/IMG_1144.JPG"
---
Infection Control and Hospital Epidemiology. Can be found [here](https://doi.org/10.1017/ice.2022.218).
[Download paper.](https://eotles.com/assets/papers/prospective_evaluation_of_data_driven_models_to_predict_daily_risk_of_clostridioides_difficile_infection_at_2-large_academic_health_centers.pdf)
## Abstract
Many data-driven patient risk stratification models have not been evaluated prospectively. We performed and compared the prospective and retrospective evaluations of 2 Clostridioides difficile infection (CDI) risk-prediction models at 2 large academic health centers, and we discuss the models’ robustness to data-set shifts.
------------------------
File: 2022-09-19-UMich-IOE-Promo-Video.md
Creation Date: "Sat, 27 Jul 2024 12:20:28 +0000"
---
title: "UMich IOE Promo Video"
categories:
- Blog
tags:
- Blog
- industrial engineering
- operations research
---
Was featured in the University of Michigan Department of Industrial and Operations Engineering promotional video.
> University of Michigan Industrial and Operations Engineering graduates are in high demand and use mathematics, and data analytics to launch their careers and create solutions across the globe in business, consulting, energy, finance, healthcare, manufacturing, robotics, aerospace, transportation, supply chain and more.
------------------------
File: 2022-11-02-Using-NLP-to-determine-factors-associated-with-high-quality-feedback.md
Creation Date: "Sat, 27 Jul 2024 12:20:28 +0000"
---
title: "Using natural language processing to determine factors associated with high‐quality feedback"
categories:
- Blog
- Research
tags:
- Blog
- Research
- medicine
- healthcare
- artificial intelligence
- machine learning
- natural language processing
- medical education
- SIMPL
header:
teaser: "/assets/images/insta/IMG_0591.JPG"
overlay_image: "/assets/images/insta/IMG_0591.JPG"
---
Global Surgical Education. Can be found [here](https://doi.org/10.1007/s44186-022-00051-y).
[Download paper.](https://eotles.com/assets/papers/using_NLP_to_determine_factors_associated_with_high_quality_feedback.pdf)
## Abstract
### Purpose
Feedback is a cornerstone of medical education. However, not all feedback that residents receive is high-quality. Natural language processing (NLP) can be used to efficiently examine the quality of large amounts of feedback. We used a validated NLP model to examine factors associated with the quality of feedback that general surgery trainees received on 24,531 workplace-based assessments of operative performance.
### Methods
We analyzed transcribed, dictated feedback from the Society for Improving Medical Professional Learning’s (SIMPL) smartphone-based app. We first applied a validated NLP model to all SIMPL evaluations that had dictated feedback, which resulted in a predicted probability that an instance of feedback was “relevant”, “specific”, and/or “corrective.” Higher predicted probabilities signaled an increased likelihood that feedback was high quality. We then used linear mixed-effects models to examine variation in predictive probabilities across programs, attending surgeons, trainees, procedures, autonomy granted, operative performance level, case complexity, and a trainee’s level of clinical training.
### Results
Linear mixed-effects modeling demonstrated that predicted probabilities, i.e., a proxy for quality, were lower as operative autonomy increased (“Passive Help” B = − 1.29, p < .001; “Supervision Only” B = − 5.53, p < 0.001). Similarly, trainees who demonstrated “Exceptional Performance” received lower quality feedback (B = − 12.50, p < 0.001). The specific procedure or trainee did not have a large effect on quality, nor did the complexity of the case or the PGY level of a trainee. The individual faculty member providing the feedback, however, had a demonstrable impact on quality with approximately 36% of the variation in quality attributable to attending surgeons.
### Conclusions
We were able to identify actionable items affecting resident feedback quality using an NLP model. Attending surgeons are the most influential factor in whether feedback is high quality. Faculty should be directly engaged in efforts to improve the overall quality of feedback that residents receive.
------------------------
File: 2022-12-20-Teaching-AI.md
Creation Date: "Sat, 27 Jul 2024 12:20:28 +0000"
---
title: "Teaching AI as a Fundamental Toolset of Medicine"
categories:
- Blog
- Research
tags:
- Blog
- Research
- medical education
- medical school
- artificial intelligence
- machine learning
header:
teaser: "/assets/images/insta/IMG_0440.JPG"
overlay_image: "/assets/images/insta/IMG_0440.JPG"
---
New article out in Cell Reports Medicine. It is a [perspective paper on incorporating AI into medical education](https://doi.org/10.1016/j.xcrm.2022.100824) with Drs. Cornelius A. James, Kimberly D. Lomis, and James Woolliscroft.
[Download paper.](https://eotles.com/assets/papers/teaching_AI_as_a_fundamental_toolset_of_medicine.pdf)
## Abstract
Artificial intelligence (AI) is transforming the practice of medicine. Systems assessing chest radiographs, pathology slides, and early warning systems embedded in electronic health records (EHRs) are becoming ubiquitous in medical practice. Despite this, medical students have minimal exposure to the concepts necessary to utilize and evaluate AI systems, leaving them under prepared for future clinical practice. We must work quickly to bolster undergraduate medical education around AI to remedy this. In this commentary, we propose that medical educators treat AI as a critical component of medical practice that is introduced early and integrated with the other core components of medical school curricula. Equipping graduating medical students with this knowledge will ensure they have the skills to solve challenges arising at the confluence of AI and medicine.
------------------------
File: 2023-01-12-STAT-News-medical-schools-missing-mark-on-AI.md
Creation Date: "Sat, 27 Jul 2024 12:20:28 +0000"
---
title: "STAT News: How medical schools are missing the mark on artificial intelligence"
categories:
- Blog
- Press
tags:
- Blog
- Press
- artificial intelligence
- machine learning
- medical education
- medical school
- STAT News
header:
teaser: "/assets/images/insta/IMG_0388.JPG"
overlay_image: "/assets/images/insta/IMG_0388.JPG"
---
Discussed my recent [perspective paper on incorporating AI into medical education](https://www.sciencedirect.com/science/article/pii/S2666379122003834) with Dr. James Woolliscroft and Katie Palmer of STAT News. Check out the full discussion [here](https://www.statnews.com/2023/01/12/medical-school-artificial-intelligence-health-curriculum/).
------------------------
File: 2023-02-22-RISE-VTC-AI-MedEd.md
Creation Date: "Sat, 27 Jul 2024 12:20:28 +0000"
---
title: "RISE Virtual Talking Circle: Innovations in Machine Learning and Artificial Intelligence for Application in Education"
categories:
- Blog
- Talk
tags:
- medicine
- machine learning
- artificial intelligence
- medical educcation
header:
teaser: "/assets/images/insta/IMG_0302.JPG"
overlay_image: "/assets/images/insta/IMG_0302.JPG"
---
University of Michigan Medical School RISE (Research. Innovation. Scholarship. Education) virtual talking circle discussion with Dr. Cornelius James.
Discussed the need for integration of AI education into undergraduate medical education (medical school). Echoed some of the findings from our [Cell Reports Medicine paper](https://www.sciencedirect.com/science/article/pii/S2666379122003834).
[Link to presentation.](https://eotles.com/assets/presentations/2023_RISE_VTC/AI_RISE_VTC.pdf)
------------------------
File: 2023-03-16-NAM-AI-HPE.md
Creation Date: "Sat, 27 Jul 2024 12:20:28 +0000"
---
title: "National Academy of Medicine: AI in Health Professions Education Workshop"
categories:
- Blog
- Talk
tags:
- medicine
- machine learning
- artificial intelligence
- medical education
- national academies
header:
teaser: "/assets/images/insta/IMG_0212.JPG"
overlay_image: "/assets/images/insta/IMG_0212.JPG"
---
Panel discussion on AI in health professions education.
I joined a panel of learners to share our perspectives on how AI should be incorporated into health professions education. Moderated by Mollie Hobensack and Dr. Cornelius James.
Panelists included: Noahlana Monzon, CPMA Nutrition Student, University of Oklahoma, Dallas Peoples, PhD Candidate in Sociology, Texas Woman's University, Winston Guo, MD Candidate, Weill Cornell Medical College, Gabrielle Robinson, PhD Student in Medical Clinical Psychology, Uniformed Services, University of the Health Sciences, Alonzo D. Turner, PhD Student, Counseling and Counselor Education, Syracuse University & 2022 NBCC Doctoral Minority Fellow and myself.
------------------------
File: 2023-03-23-html-svg-experiments.md
Creation Date: "Sat, 27 Jul 2024 12:20:28 +0000"
---
title: HTML/SVG Experiment
categories:
- Blog
tags:
- Blog
- HTML
- SVG
header:
teaser: "/assets/images/random_gradient_hello.svg"
overlay_image: "/assets/images/random_gradient_hello.svg"
---
Based on a [tutorial by Nikola Đuza](https://pragmaticpineapple.com/adding-custom-html-and-css-to-github-readme/).
------------------------
File: 2023-04-19-Collaborative-for-HFE-AI-Overview.md
Creation Date: "Sat, 27 Jul 2024 12:20:28 +0000"
---
title: "Collaborative for Human Factors, Cognitive Load, and Well-being: AI Overview"
categories:
- Blog
- Talk
tags:
- medicine
- machine learning
- artificial intelligence
- human factors engineering
- industrial engineering
- health systems engineering
- chatGPT
header:
teaser: "/assets/images/insta/IMG_0045.JPG"
overlay_image: "/assets/images/insta/IMG_0045.JPG"
excerpt: "Collaborative for Human Factors discussion on artificial intelligence, ChatGPT, and applicable research."
---
I covered foundational information about AI, its use in other domains, and potential and its perils in medicine. The rapid uptake of AI motivates an [argument for increased AI training in medical school and interprofessional education between engineers and physicians](https://www.sciencedirect.com/science/article/pii/S2666379122003834).
Additionally briefly discussed how [ChatGPT](https://chat.openai.com) functions and its potential limitations.
The recording was made after the presentation so that collaborative members could refer to it again.
## Recording
## Slides
[Link to presentation.](https://eotles.com/assets/presentations/2023_Collaborative_for_Human_Factors/20230418_AI_Overview.pdf)
------------------------
File: 2023-05-01-Hello-World-2.md
Creation Date: "Sat, 27 Jul 2024 12:20:28 +0000"
---
title: "Hello, World! 2.0"
categories:
- Blog
tags:
- blog
- software development
- web development
- blog
- chatGPT
- generative AI
- artificial intelligenc
header:
teaser: "/assets/images/insta/IMG_0289.JPG"
overlay_image: "/assets/images/insta/IMG_0289.JPG"
excerpt: "New blog, who dis?"
---
NB: this post was written by ChatGPT.
If you've been a regular reader of my blog, you might have noticed some changes around here recently. Don't worry, it's not just you! I've updated my blog to a new platform, something that's been a journey of exploration, experimentation, and ultimately, satisfaction.
Let's rewind a bit. The world of blogging platforms is vast and varied, each with its unique strengths and challenges. My goal was to find a platform that aligned with my specific needs. These were:
1. Writing posts in Markdown: As a fan of simplicity and efficiency, I wanted a platform that supported writing posts in Markdown. It's an easy-to-use syntax that streamlines the writing process and keeps the focus on the content, not the formatting.
2. Automated blog and page creation: While I appreciate the beauty of raw HTML and CSS, I wanted a platform that took care of the heavy lifting so I could focus on what I love - writing and engaging with all of you.
3. Platform independence: I didn't want my content to be locked into a specific platform. The ability to change the site in the future, if need be, was important to me.
4. Hassle-free hosting: To avoid dealing with the headaches of security and updating, I wanted a platform that didn't require me to manage my own hosting.
In my search, I tried out [Ghost](https://ghost.org), a sleek and visually appealing platform. While it was beautiful to look at, I found it to be fairly restrictive for my needs. I also experimented with [Squarespace](https://www.squarespace.com) and [Wix](https://www.wix.com), popular choices in the blogging world. However, they too fell short of my specific requirements.
After much exploration, I finally found a match in the combination of a [Jekyll blog](https://jekyllrb.com) with a [Minimal Mistakes theme](https://mmistakes.github.io/minimal-mistakes/), all hosted as a [GitHub Pages page](https://pages.github.com). It's the Goldilocks of blogging platforms for me - just right.
Jekyll, a simple, blog-aware, static site generator, checked all my boxes. It supports Markdown, automates blog and page creation, and isn't tied to a specific platform. The Minimal Mistakes theme added a layer of elegance and readability to the mix, aligning with my preference for a clean and minimalistic design. Hosting the blog as a GitHub page took care of the hosting concerns, providing a secure and updated environment for my blog.
Transitioning my old blog to this new tech stack was a learning experience, but the result has been rewarding. I'm excited about this new chapter and look forward to continuing to share my thoughts, experiences, and insights with you all.
Post script, by Erkin: I used [OpenAI's web-enabled ChatGPT4](https://chat.openai.com/?model=gpt-4-browsing) to generate this post. It first scraped my existing blog posts and then wrote the above post. Overall, I think it did a fairly good job of capturing my "conversational, detailed, and engaging" tone. I used the following prompt to generate the post:
> could you help me write a new blog post for my blog? first review some of my blog posts at https://eotles.com/blog/
>
> then write a post about my updated blog. the focus should be on the technical choice of platform. I chose to use a Minimal-Mistakes themed (https://mmistakes.github.io/minimal-mistakes/) Jekyll blog (https://jekyllrb.com) hosted as a GitHub page. I conducted a fairly exhaustive search of different blogging platforms and came to this combination as it met my requirements which where:
> 1. writing posts in markdown
> 2. automated blog and page creation - didn't want to have to write raw html or css
> 3. not having content locked into a specific platform - wanted to be able to change the site in the future - if need be
> 4. not having to deal with my own hosting - avoiding security and updating headaches
>
> I tried https://ghost.org which was very pretty but was fairly restrictive and I tried square space and wix. Eventually I settled on this tech stack and converted my old blog to this one
------------------------
File: 2023-07-22-Updating-Clinical-Risk-Stratification-Models-Using-Rank-Based-Compatibility.md
Creation Date: "Sat, 27 Jul 2024 12:20:28 +0000"
---
title: "Updating Clinical Risk Stratification Models Using Rank-Based Compatibility"
categories:
- Blog
- Research
tags:
- Blog
- Research
- early warning system
- medicine
- healthcare
- artificial intelligence
- machine learning
- updating
- Anthropic
header:
teaser: "/assets/images/insta/6D2D87B6-7406-43F5-A6B9-FC06FCFEED36.jpg"
overlay_image: "/assets/images/insta/6D2D87B6-7406-43F5-A6B9-FC06FCFEED36.jpg"
excerpt: "As machine learning models become more integrated into clinical care, how can we update them without violating user expectations? We proposed a new rank-based compatibility measure and loss function to develop clinical AI that better aligns with physician mental models. High rank-based compatibility is not guaranteed but can be achieved through optimization, our approach yields updated models that better meet user expectations, promoting clinician-model team performance."
---
Check out our new paper: [Updating Clinical Risk Stratification Models Using Rank-Based Compatibility: Approaches for Evaluating and Optimizing Joint Clinician-Model Team Performance](https://www.mlforhc.org/s/ID103_Research-Paper_2023.pdf).
It was accepted to the 2023 [Machine Learning for Healthcare Conference](https://www.mlforhc.org/#).
[Download paper.](https://eotles.com/assets/papers/2023_MLHC_rank_based_compatibility.pdf)
[Paper on arXiv.](https://arxiv.org/abs/2308.05619)
Code for the new measure, loss function, and experimental analysis can be found at [this GitHub repo](https://github.com/eotles/MLHC_2023_rank_based_compatibility_supplemental_code).
## Abstract
As data shift or new data become available, updating clinical machine learning models may be necessary to maintain or improve performance over time. However, updating a model can introduce compatibility issues when the behavior of the updated model does not align with user expectations, resulting in poor user-model team performance. Existing compatibility measures depend on model decision thresholds, limiting their applicability in settings where models are used to generate rankings based on estimated risk. To address this limitation, we propose a novel rank-based compatibility measure, $$C^R$$, and a new loss function that optimizes discriminative performance while encouraging good compatibility. Applied to a case study in mortality risk stratification leveraging data from MIMIC, our approach yields more compatible models while maintaining discriminative performance compared to existing model selection techniques, with an increase in $$C^R$$ of $$0.019$$ ($$95\%$$ confidence interval: $$0.005$$, $$0.035$$). This work provides new tools to analyze and update risk stratification models used in settings where rankings inform clinical care.
Here's a 30,000 foot summary of the paper.
## Updating Clinical Risk Models While Maintaining User Trust
As machine learning models become more integrated into clinical care, it's crucial we understand how updating these models impacts end users. Models may need to be retrained on new data to maintain predictive performance. But if updated models behave differently than expected, it could negatively impact how clinicians use them.
My doctoral advisors (Dr. Brian T. Denton and Dr. Jenna Wiens) and I recently explored this challenge of updating for clinical risk stratification models. These models estimate a patient's risk of some outcome, like mortality or sepsis. They're used to identify high-risk patients who may need intervention.
### Backwards Trust Compatibility
An existing compatibility measure is [backwards trust compatibility (developed by Bansal et al.)](https://ojs.aaai.org/index.php/AAAI/article/view/4087). It checks if the original and updated models label patients correctly in the same way. But it depends on setting a decision "threshold" to convert risk scores into labels.
In many clinical settings, like ICUs, physicians may use risk scores directly without thresholds. So we wanted a compatibility measure that works for continuous risk estimates, not just thresholded labels.
### Rank-Based Compatibility
We introduced a new rank-based compatibility measure. It doesn't require thresholds. Instead, it checks if the updated model ranks patients in the same order as the original model.
For example, if the original model ranked patient A's risk higher than patient B, does the updated model preserve this ordering? The more patient pair orderings it preserves, the higher its rank-based compatibility.
### Training Models to Prioritize Compatibility
But simply measuring compatibility isn't enough - we want to optimize it during model training. So we proposed a new loss function that balances predictive performance with rank-based compatibility.
Using a mortality prediction dataset, we compared models trained normally vs with our compatibility-aware loss function. The optimized models achieved significantly better compatibility without sacrificing much accuracy.
### Why This Matters
Model updating is inevitable as new data emerge. But unintended changes in model behavior can violate user expectations. By considering compatibility explicitly, we can develop clinical AI that better aligns with physician mental models.
This helps ensure updated models are readily adopted, instead of met with skepticism. It's a small but important step as we integrate machine learning into high-stakes medical settings. We're excited to continue improving these models collaboratively with end users.
Please let me know if you have any questions.
Cheers,
Erkin
[Go ÖN Home](https://eotles.com)
N.B. this blog post was writen in collaboration with [Anthropic's Claude](https://www.anthropic.com).
------------------------
File: 2023-07-22-qr-code-generator.md
Creation Date: "Sat, 27 Jul 2024 12:20:28 +0000"
---
title: "QR Code Generator"
categories:
- Blog
tags:
- Blog
- QR Code
- javascript
---
A simple QR code generator that you can use to make QR code embeded with the strings of your dreams!
I made this for a series of presentations I gave.
It enabled me to make a QR code quickly from a URL (usually from this site) without having to google and find a website to do this.
I had ChatGPT write up the javascript, which was pretty slick.
Note.
This tool is entirely for me.
If you get use out of it too, nice!
QR Code Generator by eotlesDownload QR Code
------------------------
File: 2023-07-25-INFORMS-Healthcare-2023.md
Creation Date: "Sat, 27 Jul 2024 12:20:28 +0000"
---
title: "INFORMS Healthcare: Rank-based Compatibility"
categories:
- Blog
- Talk
tags:
- INFORMS
- industrial engineering
- operations research
- medicine
- healthcare
- research
- machine learning
- artificial intelligence
header:
teaser: "/assets/images/insta/IMG_0442.JPG"
overlay_image: "/assets/images/insta/IMG_0442.JPG"
---
Presentation at INFORMS Healthcare 2023 on our work on rank-based compatibility.
You can find a link to the post about the upcoming paper [here](https://eotles.com/blog/research/Updating-Clinical-Risk-Stratification-Models-Using-Rank-Based-Compatibility/).
View a copy of the presentation slides below.
[Link to download presentation.](https://eotles.com/assets/presentations/2023_INFORMS_Healthcare/202300726_INFORMS_healthcare_rank_based_compatibility.pdf)
A recording of this presentation can be found here.
## Abstract
Updating clinical machine learning models is necessary to maintain performance, but may cause compatibility issues, affecting user-model interaction. Current compatibility measures have limitations, especially where models generate risk-based rankings. We propose a new rank-based compatibility measure and loss function that optimizes discriminative performance while promoting good compatibility. We applied this to a mortality risk stratification study using MIMIC data, resulting in more compatible models while maintaining performance. These techniques provide new approaches for updating risk stratification models in clinical settings.
------------------------
File: 2023-07-26-hangman.md
Creation Date: "Sat, 27 Jul 2024 12:20:28 +0000"
---
title: "Hangman"
categories:
- Blog
tags:
- Blog
- game
- javascript
---
A simple hangman game. Made with chatGPT.
Hangman Game
Hangman Game
Incorrect guesses:
------------------------
File: 2023-08-11-Machine-Learning-for-Healthcare-2023.md
Creation Date: "Sat, 27 Jul 2024 12:20:28 +0000"
---
title: "2023 Machine Learning for Healthcare Conference"
categories:
- Blog
- Talk
tags:
- Machine Learning for Healthcare Conference
- medicine
- healthcare
- research
- machine learning
- artificial intelligence
header:
teaser: "/assets/images/insta/E35BD8D3-0BE7-4D05-BDD7-C42C47F7C487.jpg"
overlay_image: "/assets/images/insta/E35BD8D3-0BE7-4D05-BDD7-C42C47F7C487.jpg"
---
Presentation at Machine Learning for Healthcare 2023 in New York on our work on rank-based compatibility.
During the conference I presented a brief spotlight talk introducing our work and also had the chance to present a poster going into more detail.
I've included copies of both in this blog post.
You can find a link to the post about the paper [here](https://eotles.com/blog/research/Updating-Clinical-Risk-Stratification-Models-Using-Rank-Based-Compatibility/).
A recording of the spotlight intro video.
Spotlight presentation slides
[Link to download presentation.](https://eotles.com/assets/presentations/2023_MLHC/20230811_MLHC_rank_based_compatibility.pdf)
Poster
[Link to download poster.](https://eotles.com/assets/presentations/2023_MLHC/2023_MLHC_poster_20230809.pdf)
## Abstract
Updating clinical machine learning models is necessary to maintain performance, but may cause compatibility issues, affecting user-model interaction. Current compatibility measures have limitations, especially where models generate risk-based rankings. We propose a new rank-based compatibility measure and loss function that optimizes discriminative performance while promoting good compatibility. We applied this to a mortality risk stratification study using MIMIC data, resulting in more compatible models while maintaining performance. These techniques provide new approaches for updating risk stratification models in clinical settings.
------------------------
File: 2023-09-12-Github-Action-for-Post-Concatenation.md
Creation Date: "Sat, 27 Jul 2024 12:20:28 +0000"
---
title: "It's Automation All the Way Down! How to Use GitHub Actions for Blogging Automation with LLMs"
last_modified_at: 2023-12-08
categories:
- Blog
tags:
- git
- github
- github actions
- github pages
- CI/CD
- blogging
- jekyll
- minimal mistakes
- minimal-mistakes
- automation tools
- web development
- workflow optimization
- LLM
- chatGPT
- data engineering
header:
teaser: "/assets/images/insta/IMG_2253.JPG"
overlay_image: "/assets/images/insta/IMG_2253.JPG"
overlay_filter: 0.5 # same as adding an opacity of 0.5 to a black background
excerpt: "CI/CD automation isn't just for large-scale projects; it's a game-changer for individual programmers. I've started using the power of GitHub Actions to improve my blogging process, making it more efficient. I ❤️ Automation."
---
# The LLM Advantage in Blogging
I've used [large language model (LLM)](https://en.wikipedia.org/wiki/Large_language_model) powered chatbots ([ChatGPT](https://chat.openai.com) & [Claude](https://claude.ai/chats) to help with some of my writing. They've been especially beneficial with blog posts where I have functionality dependent on JavaScript code.
# The Automation Dilemma
Utilizing these LLM chatbots is pretty straightforward, but it gets annoying when you want to provide them with writing samples. You can pick and choose a couple representative posts and share those, but that's too scattershot for me. Ideally, I'd like my whole corpus of blog posts to be used as samples for the chatbots to draw from. I had written some python scripts that loop over my posts and create a concatenated file. This worked fine for creating a file - but it was annoying to manually kick off the process every time I made a new post. So, I started thinking about how to automate the process.
There are many ways to approach it, but I wanted to keep it simple. The most straightforward route was to build off my existing automation infrastructure - the GitHub pages build process.
# GitHub Actions: My Automation Hero
The GitHub pages build process automatically converts the documents I use to write my blog (markdown files) into the web pages you see (HTML). GitHub provides this service as a tool for developers to quickly spin up webpages using the [GitHub Actions](https://github.com/features/actions) framework. GitHub actions are fantastic as they enable [continuous integration and continuous delivery/deployment (CI/CD)](https://en.wikipedia.org/wiki/CI/CD).
graph TB
%% Primary Path
A[Push new blog .md post to github] --> BA
BB --> CA
CB --> D[Commit & push changes]
%% GitHub Pages Build Process
subgraph B[GitHub Pages Build Process]
BA[Build eotles.com webpages] --> BB[Trigger: gh-pages branch]
end
%% Concatenate .md Files Action
subgraph C[Concatenate .md Files Action]
CA[Create file] --> CB[Loop over all posts and concat to file]
end
%% .md Files
A -.-> P[.md files]
P -.-> B
P -.-> C